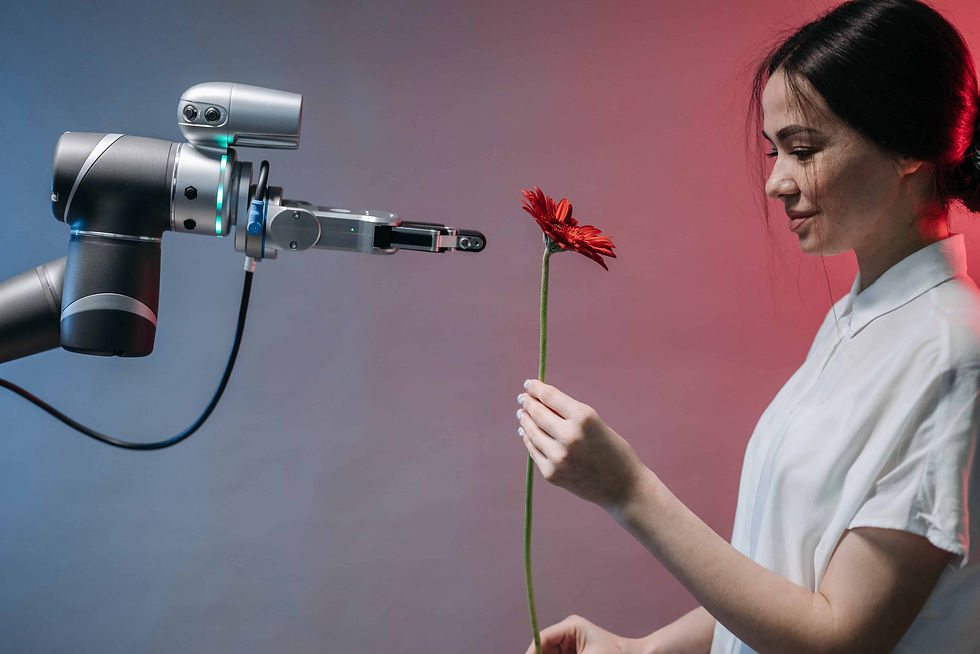
I. Introduction
1.1. Brief on AI and Edge Computing: Definitions and Basic Understanding
1.2. Relevance of AI and Edge Computing in Today’s Manufacturing World
2.1. Historical Perspective: From Industry 1.0 to 4.0
2.2. The Emergence and Growth of AI and Edge Computing in Manufacturing
3.1. How AI is Shaping Manufacturing
3.2. Edge Computing: An Enabler of Industrial IoT
3.3. The Convergence of AI and Edge Computing
4.1. Improved Efficiency and Productivity
4.2. Enhanced Quality Control
4.3. Predictive Maintenance and Downtime Reduction
4.4. Safer Work Environments
4.5. Supply Chain Optimization
5.1. AI and Edge Computing Success Stories in Manufacturing
5.2. Highlighting Industry Pioneers and Their Approaches
6.1. Technical Challenges and Solution Strategies
6.2. Security Risks and Mitigation Measures
6.3. Ethical Considerations and Regulations
7.1. Predicted Technological Advancements
7.2. Role of AI and Edge Computing in Sustainable Manufacturing
7.3. Preparing for the Future: Skills, Training, and Workforce Development
VIII. Conclusion
8.1. Summary of Key Points
8.2. The Future Manufacturing Landscape with AI and Edge Computing
IX. FAQs
AI and edge computing in manufacturing 2024: Represent the future.
I. Introduction
Our journey begins with understanding the concepts of Artificial Intelligence (AI) and edge computing. Imagine these technologies as the brain and nervous system of modern factories, respectively. AI, with its capability for predictive analytics and Machine Learning (ML), has turned into a game-changer for demand forecasting and decision-making in the manufacturing industry. Coupled with edge computing's proficiency for real-time data processing, it improves the industry's operational efficiency and productivity significantly. With AI and edge computing at the helm, we witness a revolution in supply chain logistics, planning and management, paving the way for higher levels of automation and robotics in the manufacturing processes.
Key Aspects to Look Out For
Understanding the role of AI and edge computing in the digital transformation of the manufacturing industry.
The impact of predictive analytics and ML in demand forecasting and decision-making.
The significance of edge computing in improving real-time operational efficiency and productivity.
The revolution in supply chain logistics, planning and management facilitated by AI and edge computing.
The growth of automation and robotics in manufacturing processes powered by AI and edge computing.
II. Evolution of AI and Edge Computing in Manufacturing
Tracing the path of evolution from steam engines to AI-driven smart factories, we witness how industries have morphed from mechanization to digitization. Automation, powered by robotics, transformed the face of manufacturing. This evolution heralded the breakthrough of AI and edge computing, marking a new era in the industry. As AI strategies became more sophisticated, similar to a master chess player anticipating moves and adapting to changing scenarios, the reliance on these technologies in manufacturing processes grew exponentially. This growth is driven by the need for improved logistics, streamlined planning, optimized scheduling, and the rise of autonomous systems. However, the increased use of AI also ushers in ethical considerations for ensuring its responsible utilization in manufacturing.
Key Aspects to Look Out For
The journey of manufacturing evolution from mechanization to digitization, culminating in the breakthrough of AI and edge computing.
The role of automation and robotics in transforming manufacturing.
The rise and growth trends of AI and edge computing adoption in the manufacturing industry.
The driving forces behind this growth: improved logistics, streamlined planning, optimized scheduling, and the advent of autonomous systems.
Ethical considerations and challenges in the wake of increased AI usage in manufacturing.
III. Role of AI and Edge Computing in Modern Manufacturing
Think of AI and edge computing as the two pillars supporting the grand edifice of modern manufacturing. As AI shapes the manufacturing landscape with its intelligent decision-making capabilities, edge computing boosts the functionality of Industrial IoT, leading to a new era of interconnected, smart manufacturing systems. The convergence of these two technologies is akin to the meeting of the mind and the muscle, providing both the intelligence and the power required for enhanced manufacturing processes.
3.1. How AI is Shaping Manufacturing
AI, with its prowess in ML and data analytics, is progressively shaping manufacturing. It's like the architect, meticulously planning, predicting, and optimizing the manufacturing process. It plays a pivotal role in enhancing supply chain efficiency, improving logistics, and streamlining operations. From demand forecasting to production planning, AI is introducing levels of precision and efficiency never seen before.
Main Ideas
AI's role in enhancing supply chain efficiency and improving logistics.
The impact of AI on streamlining operations and demand forecasting.
The contribution of AI to production planning.
3.2. Edge Computing: An Enabler of Industrial IoT
As AI works on planning and optimizing, edge computing provides the means to execute these plans with minimal latency. It's like the nervous system of the smart factory, rapidly transmitting data for real-time decision-making. By enabling Industrial IoT, edge computing facilitates efficient communication between machines, leading to autonomous systems and robotics.
Main Ideas
The role of edge computing in facilitating real-time decision-making.
How edge computing enables efficient machine-to-machine communication.
The contribution of edge computing to the rise of autonomous systems and robotics.
3.3. The Convergence of AI and Edge Computing
The convergence of AI and edge computing in manufacturing is a marriage of intellect and execution. Like the interplay between a maestro and musicians in an orchestra, AI conducts the symphony of production processes while edge computing ensures each instrument, or machine, performs its part at the right time.
Main Ideas
How AI and edge computing work together in the manufacturing process.
The synergistic effects of the convergence of AI and edge computing.
The role of this convergence in the orchestration of production processes.
Quick Facts
AI can reduce forecasting errors by up to 50% and reduce lost sales by 65% with better product availability.
Edge computing can reduce a factory's data transport time and costs, improve scalability, and secure data privacy.
The combination of AI and edge computing can increase production efficiency by up to 20%.
By 2024, it's estimated that over 50% of new enterprise IT infrastructure deployed will be at the edge rather than corporate data centers, up from less than 10% today.
By 2024, over 50% of data from IoT will be processed at the edge, as estimated by IDC.
Table: Examples of User Experiences and Corresponding Right Paths
User Experience | Right Path |
Difficulty in managing supply chain and logistics. | Implement AI for demand forecasting, inventory management, and optimizing logistics. |
Latency in data processing hindering real-time decision making. | Adopt edge computing for rapid data transmission and real-time analytics. |
Inefficiencies in machine-to-machine communication. | Utilize edge computing to enable Industrial IoT and improve machine interoperability. |
Errors and inefficiencies in production planning. | Leverage AI for precise production planning and optimization. |
Inability to handle large volumes of data generated by IoT devices. | Implement edge computing to process IoT data at the source, improving speed and reducing bandwidth usage. |
IV Transformative Benefits of AI and Edge Computing in Manufacturing
Artificial Intelligence (AI) and Machine Learning (ML) are increasingly transforming the world of manufacturing and supply chain. The integration of AI-powered logistics and distribution systems and ML for supply chain optimization are enabling an intelligent supply chain solution that boosts efficiency and productivity.
4.1 Improved Efficiency and Productivity
Automation in manufacturing and logistics through AI and robotics has led to significant improvements in efficiency and productivity. The use of autonomous systems in the industry has streamlined operations, ensuring seamless planning and scheduling. These AI-driven systems offer smart inventory control, facilitating a well-optimized production process.
Example Tool: An AI-powered automation tool like 'Cognite' for autonomous operations.
Cognite Data Fusion is a tool that unifies industrial data to power operational insight and AI applications. It can help automate production processes and increase efficiency.
4.2 Enhanced Quality Control
AI and ML have revolutionized quality control in manufacturing. Data-driven decision-making, facilitated by analytics and insights from AI, has led to a reduction in errors, enhancing the overall quality of products.
Example Tool: 'Google's Cloud AutoML' for quality inspection.
Google's Cloud AutoML. This suite of machine learning products lets developers with limited machine learning expertise train high-quality models. It can be used for image recognition tasks, essential for identifying defects and ensuring quality in the manufacturing process.
4.3 Predictive Maintenance and Downtime Reduction
Predictive analytics for demand forecasting has become an essential tool in the industry. AI systems can predict when a machine is likely to fail, allowing for timely maintenance and reduced downtime.
Example Tool: 'IBM's Watson' for predictive maintenance.
IBM's Watson for Manufacturing. Watson leverages AI to learn from historical maintenance data, predict failures, and schedule maintenance ahead of time, minimizing downtime.
4.4 Safer Work Environments
Robotics and AI in warehouse management have not only increased productivity but have also ensured safer work environments. Autonomous robots can take over hazardous tasks, reducing the risk of accidents.
Example Tool: 'Boston Dynamics' Spot robot' for inspecting potentially dangerous areas.
Boston Dynamics' Spot robot. The Spot robot is a nimble mobile robot that can navigate environments, even hazardous ones, with ease, helping maintain a safer work environment by performing tasks in places that may pose risks to humans.
4.5 Supply Chain Optimization
AI's role in supply chain optimization cannot be overstated. With predictive demand forecasting, distribution can be effectively managed. Digital transformation in manufacturing through AI is improving logistics, making the supply chain more responsive and efficient.
Example Tool: 'SAP's Integrated Business Planning' for supply chain optimization.
SAP's Integrated Business Planning (IBP). SAP IBP is a supply chain planning tool that provides demand-driven sales, inventory, and operations planning. It can help organizations anticipate and respond to dynamic market conditions.
Table:
Objective | Action | Key Performance Indicator (KPI) | Example Tool |
Improve Efficiency & Productivity | Implement AI-powered automation systems for autonomous operations | Increase in operational throughput | Cognite |
Enhance Quality Control | Use AI and ML for data-driven decision making and quality inspection | Reduction in product defects | Google's Cloud AutoML |
Reduce Downtime | Utilize AI for predictive maintenance | Reduction in machinery downtime | IBM's Watson |
Ensure Safer Work Environment | Introduce AI and Robotics in warehouse management for potentially dangerous tasks | Decrease in workplace accidents | Boston Dynamics' Spot robot |
Optimize Supply Chain | Implement AI in demand forecasting for effective distribution management | Increase in supply chain responsiveness | SAP's Integrated Business Planning |
Tips and Tricks
Start Small, Scale Later: Begin by automating small, non-critical tasks. Once you understand how AI works and its impact, you can scale to bigger projects.
Align with Business Goals: Always ensure your AI initiatives align with your overall business goals and strategies. This helps ensure the technologies you implement provide actual value to your organization.
Focus on Data Quality: AI and ML are as good as the data they're trained on. Pay attention to data quality and consider using data cleaning tools.
Invest in Training: Make sure your workforce is well-trained and ready to work alongside AI and robotics. This can involve workshops or working with an experienced partner.
Plan for Change Management: Implementing AI and robotics often entails changes in work processes. Make sure to have a solid change management plan in place to ensure a smooth transition.
V. Real-world Examples and Case Studies
5.1. AI and Edge Computing Success Stories in Manufacturing
Artificial intelligence (AI) and edge computing have brought about transformative changes in the manufacturing sector.
Toyota: Toyota's use of AI in production processes has led to increased efficiency and reduced defects. Employees report smoother operations, less time spent on identifying and correcting errors, and an overall increase in job satisfaction.
Coca-Cola: By integrating AI and machine learning for supply chain optimization, Coca-Cola has significantly improved their inventory management. Distributors report better coordination, more accurate demand forecasting, and improved overall business relationships.
Siemens: Siemens has leveraged AI in its manufacturing operations to predict machinery maintenance needs before they result in downtime. This has resulted in higher productivity levels, reduced costs, and increased customer satisfaction due to timely deliveries.
GE Appliances: By automating its manufacturing and logistics operations using AI, GE has been able to reduce its operational costs significantly. Customers report improved product quality and faster delivery times.
Nestle: Nestle's use of AI for demand forecasting and planning management has led to more accurate production planning. Retailers report better stock availability, and consumers enjoy more consistent product availability.
5.2. Highlighting Industry Pioneers and Their Approaches
Several industry pioneers are leading the way in the integration of AI and edge computing in manufacturing.
Microsoft: Microsoft's use of AI in production processes has led to significant improvements in product quality and reduction in defects. Users report higher satisfaction due to fewer product issues.
Amazon: Amazon's use of AI-powered logistics and distribution, along with robotics in warehouse management, has improved order fulfillment speed and accuracy. Customers frequently praise the company's rapid and reliable delivery.
Bosch: Bosch has implemented AI for predictive maintenance in their manufacturing facilities, leading to fewer machinery breakdowns and disruptions. Clients report improved trust in the company due to consistent production output.
IBM: Through its Watson platform, IBM has helped numerous manufacturing companies optimize their operations using AI. Users of Watson have reported improvements in productivity, efficiency, and forecasting accuracy.
Google: Through its cloud-based AI solutions, Google has supported manufacturers in improving their production processes. Clients report enhanced operational efficiency, improved quality control, and smarter inventory control.
VI. Challenges and Risks in Implementing AI and Edge Computing
Incorporating AI and edge computing into manufacturing also has its set of challenges.
6.1. Technical Challenges and Solution Strategies
Some of the technical challenges include a lack of skilled personnel, high initial implementation costs, and complex data integration. Solution strategies might involve collaborations with AI experts, investing in training for existing staff, and phased implementation approaches for better data management.
6.2. Security Risks and Mitigation Measures
Potential security risks associated with AI and edge computing include data breaches and cyber-attacks. Organizations must establish strong security protocols, conduct regular system audits, and ensure data encryption to mitigate these risks.
6.3. Ethical Considerations and Regulations
When dealing with AI and machine learning, ethical considerations around data privacy, transparency, and potential job losses cannot be overlooked. It is crucial for businesses to follow AI ethics guidelines, adhere to data privacy regulations, and consider employee reskilling programs.
Table:
Main Idea | Current State | Future Developments | Potential Challenges |
Technical Challenges and Solution Strategies | Skilled personnel shortage, complex data integration and high initial implementation costs | AI and edge computing to become more accessible and user-friendly | Ensuring a steady supply of skilled workforce, managing costs, and handling data |
Security Risks and Mitigation Measures | Increasing cyber threats, data breaches | Stronger security protocols, advanced AI-based cybersecurity solutions | Keeping up with evolving cyber threats, protecting sensitive data |
Ethical Considerations and Regulations | Ethical concerns around job displacement, data privacy and transparency | Fair and ethical AI guidelines to be widely adopted and regulated | Compliance with ethical standards and regulations, ensuring data privacy and handling job displacement |
VII. Future Trends: AI and Edge Computing in Manufacturing Beyond 2024
7.1. Predicted Technological Advancements
As we move beyond 2024, AI and edge computing are set to revolutionize the manufacturing industry even further. Here's a sneak peek at some of the predicted advancements:
AI-powered predictive maintenance: By leveraging AI and data analytics, factories will be able to predict equipment failures before they occur, minimizing downtime, and saving costs.
Autonomous robotics: Robotics will become increasingly autonomous, thanks to AI. They'll handle more complex tasks, improving productivity, and efficiency.
Smart inventory control with AI: AI will optimize inventory control, ensuring just-in-time deliveries and minimizing storage costs.
Intelligent supply chain solutions: AI will help streamline supply chains, leading to reduced waste and more sustainable practices.
Here are five user experiences to consider for these advancements:
A production manager using an AI-powered system could receive alerts about potential equipment failures, allowing them to schedule maintenance before the issue becomes a problem.
Warehouse workers might find their workload reduced as autonomous robots take over more complex, time-consuming tasks.
Inventory managers will see real-time updates about their stock levels, and they'll receive alerts when stock is low or in excess. This will help them maintain an optimal inventory level at all times.
Supply chain managers will be able to track their goods in real-time and receive updates about potential disruptions, allowing them to take action before it affects their operations.
Sustainability officers will appreciate the reduced waste from optimized supply chains, helping their companies meet their environmental targets.
7.2. Role of AI and Edge Computing in Sustainable Manufacturing
AI and edge computing will play a significant role in promoting sustainable manufacturing. They'll help reduce waste, optimize energy use, and support the shift towards circular economy practices.
Here are five user experiences to consider:
Factory managers could use AI to optimize energy use in their facilities, reducing their carbon footprint and saving on energy costs.
Quality control teams will benefit from AI's ability to detect defects early in the production process, reducing waste from faulty products.
Logistics managers will see their delivery routes optimized by AI, leading to lower fuel consumption and reduced emissions.
Resource managers could use AI to monitor and reduce water usage in their factories.
CEOs and business leaders will see their companies make significant strides towards their sustainability goals with the help of AI and edge computing.
7.3. Preparing for the Future: Skills, Training, and Workforce Development
As AI and edge computing become more prevalent, there's a need for skills and training to keep pace. Manufacturing firms must invest in workforce development to ensure their employees can leverage these technologies effectively.
Here are five user experiences to consider:
Factory workers will need training to operate and maintain new AI-powered equipment and autonomous robots.
IT staff will need to upskill to manage and secure the increased data generated by AI and edge computing.
Managers will need to understand how AI can support their decision-making and be comfortable using data-driven insights in their planning.
HR teams will need to recruit and retain staff with the necessary AI and edge computing skills.
Company leaders will need to champion the use of AI and edge computing, driving their adoption throughout the organization.
Table:
Main Idea | Current State | Future Developments | Potential Challenges |
Predicted Technological Advancements | Growing use of AI and edge computing in manufacturing | More widespread and advanced use of these technologies | Managing the pace of change, upskilling staff |
Role of AI and Edge Computing in Sustainable Manufacturing | Emerging use of AI for sustainability | AI and edge computing to become key drivers of sustainable manufacturing | Proving the ROI of sustainability initiatives |
Preparing for the Future: Skills, Training, and Workforce Development | Current skills gaps in AI and edge computing | Greater need for these skills as the technologies become more prevalent | Attracting and retaining talent, providing effective training |
VIII. Conclusion
8.1. Summary of Key Points
This article has provided a comprehensive overview of the role and impact of artificial intelligence (AI) and edge computing in the manufacturing industry. We've looked at real-world examples and case studies, revealing the successes and challenges encountered by pioneers in this field. From Toyota's increased efficiency to Coca-Cola's inventory management transformation, we've seen how AI can enhance operations and customer experiences.
We've also examined the transformative benefits of AI, including improved efficiency and productivity, enhanced quality control, predictive maintenance, and supply chain optimization. The integration of AI into production and logistic processes is more than a trend—it's a game-changer for the future of manufacturing.
Furthermore, we've delved into the challenges and risks involved in implementing these technologies. Recognizing these obstacles, such as technical challenges, security risks, and ethical considerations, is essential for manufacturers who want to remain competitive and innovative.
8.2. The Future Manufacturing Landscape with AI and Edge Computing
Looking ahead, AI and edge computing are set to further transform the manufacturing landscape. Predicted technological advancements like AI-powered predictive maintenance, autonomous robotics, and intelligent supply chain solutions hold exciting potential. At the same time, these technologies will also play a significant role in promoting sustainable manufacturing by reducing waste, optimizing energy use, and supporting circular economy practices.
But with these advancements comes the need for skills and training. Businesses must invest in workforce development to harness the power of these technologies effectively.
Key Takeaways
The key takeaways from this article are:
The transformative power of AI and edge computing: These technologies are reshaping the manufacturing industry, enhancing efficiency, productivity, and customer experiences.
Successes and challenges of AI adoption: Real-world examples demonstrate the potential of these technologies, while also highlighting the need to manage the challenges and risks associated with their implementation.
The future of manufacturing with AI and edge computing: Predicted advancements suggest an exciting future for manufacturing. The shift towards more sustainable practices will be aided by these technologies.
The importance of skills and training: As AI and edge computing become more prevalent, the need for upskilling the workforce becomes increasingly crucial.
The future of manufacturing lies in the hands of AI and edge computing. Businesses that embrace these technologies, manage the associated risks, and invest in their workforce's skills will be well-positioned to lead in the new era of manufacturing.
IX. FAQs
What is the difference between edge computing and cloud computing in manufacturing?
Edge computing and cloud computing are both critical technologies in modern manufacturing. The main difference lies in where data processing occurs. Edge computing processes data near its source (like a machine on the factory floor), reducing latency and improving efficiency. Cloud computing, on the other hand, involves sending data to a centralized server for processing. While cloud computing offers massive storage and processing power, edge computing offers speed and real-time insights, key to operational efficiency in the manufacturing process.
How does AI and edge computing contribute to the concept of smart factories?
Are there any limitations to the application of AI and edge computing in manufacturing?
What kind of jobs might be created or lost in the manufacturing industry due to AI and edge computing?
How can a manufacturing company begin implementing AI and edge computing technologies?
What role does AI play in supply chain optimization?
How does AI contribute to sustainable manufacturing?
What is the impact of AI and edge computing on workforce development?
What ethical considerations come with AI and edge computing?
What are the predicted technological advancements in AI and edge computing for manufacturing?
Comments