Maximize Data Impact: Best Budget AI Analytics Tools in 2024
- Shailesh Gowda
- Jul 9, 2024
- 21 min read
Updated: Aug 24, 2024
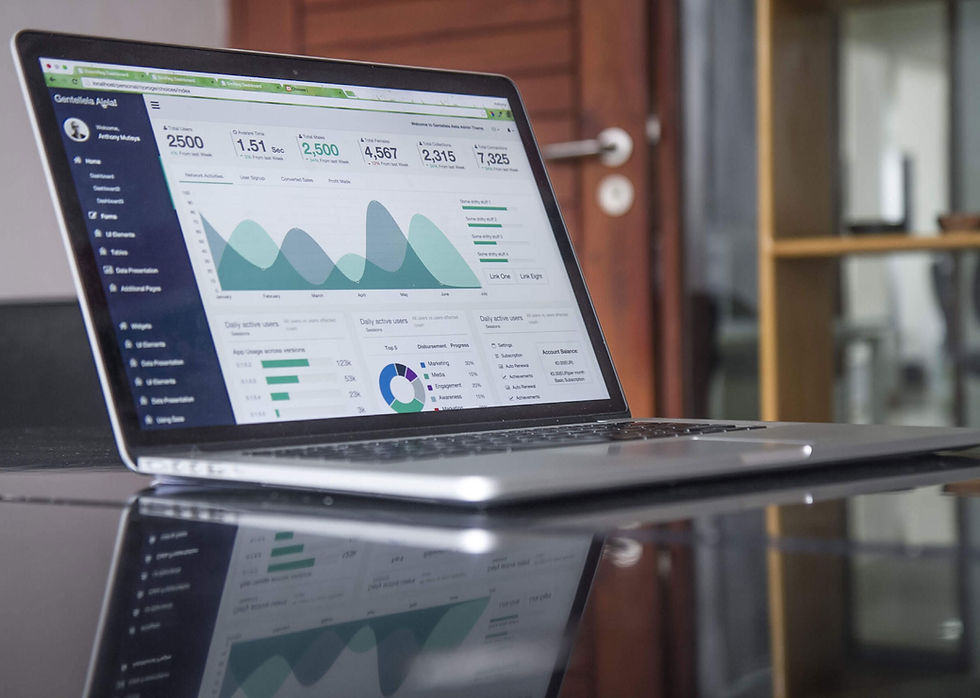
I. Introduction
A. The importance of AI in today's digital world
B. The rising demand for budget AI analytics tools
A. Explanation of AI analytics
B. The role of AI analytics in businesses
C. The impact of budget constraints on AI adoption
A. Identifying your data analysis requirements
B. Understanding the impact of your business size and type
C. Importance of scalability in choosing a tool
A. How price influences quality in AI analytics
B. Possible trade-offs when opting for budget tools
A. Brief overview of each tool
A. Detailed features
B. Pricing structure
C. Case study of successful usage
VII. Deep Dive: OpenRefine
A. Detailed features
B. Pricing structure
C. Case study of successful usage
VIII. Deep Dive: RapidMiner
A. Detailed features
B. Pricing structure
C. Case study of successful usage
A. Detailed features
B. Pricing structure
C. Case study of successful usage
X. Deep Dive: KNIME
A. Detailed features
B. Pricing structure
C. Case study of successful usage
A. Comparison of features
B. Comparison of pricing structures
C. Determining the best value for money
A. Quick overview of other options
A. Steps to adopting an AI analytics tool
B. Common pitfalls to avoid during implementation
A. Trends to watch in the AI analytics market
B. How these trends can impact the future of budget tools
XV. Conclusion
Democratizing Data: The Top Budget AI Analytics Tools in 2024
I. Introduction: The Dawn of Artificial Intelligence and Its Modern Implications
In an era where artificial intelligence (AI) and technology rule the roost, understanding the role of AI becomes imperative. AI, a concept coined in 1956, has transitioned from the realm of science fiction to a critical component of the contemporary digital world. As we dive into the complex world of AI, language models, and chatbots, let's take a moment to comprehend the significance of AI in our lives today.
A. The Importance of AI in Today's Digital World
With AI-powered programs such as GPT-3 and its more advanced sibling, GPT-4, we've seen a dramatic expansion in conversation capabilities. AI chatbots like ChatGPT, developed by OpenAI, are not only transforming the way we access the internet but also reshaping how we interact with machines. They are no longer just tools; they've become intelligent companions that aid us in everyday tasks. This democratization of AI has led to the proliferation of various AI models, with different specialties, supported by tech giants like Microsoft and Google.
ChatGPT: A product of OpenAI, ChatGPT is a robust conversational AI that employs GPT-3.5 and GPT-4, setting the bar high for AI chatbots. This advanced LLM model is a leader in the GPT series.
YouChat: This alternative chatbot, while readily available, sometimes lacks capacity blocks that make it a secondary choice to ChatGPT.
Jasper: This AI chatbot is specifically designed for businesses and marketers, offering useful writing templates and copyediting features.
Chatsonic: Supported by Google, Chatsonic is an AI chatbot tailored for news content creators, keeping them up-to-date.
Google Bard: A powerful AI chatbot for writing assistance, Google Bard is a more lightweight version of the LaMDA model from Google.
B. The Rising Demand for Budget AI Analytics Tools
As AI continues to evolve, there's an increasing demand for budget-friendly AI analytics tools. These tools, packed with features such as language model systems, NLP-driven chatbots, and more, democratize data, making it accessible to a wider audience.
Perplexity AI: Offers prompt ideation services using GPT-3 and GPT-4, perfect for content creators on a budget.
Socratic: This AI chatbot offers educational and fun graphics, making it an ideal choice for students.
Ink: An AI chatbot specifically designed for SEO and content optimization for search engines, Ink is a valuable tool for digital marketers.
Ryter: This AI chatbot streamlines the writing process for professionals, simplifying content creation.
HubSpot Chat Builder: Part of the HubSpot CRM software, this tool allows users to build their chatbots, perfect for brands wanting to deliver personalized experiences to their customers.
II. Understanding AI Analytics
A. Explanation of AI Analytics
Artificial intelligence (AI) analytics leverages machine learning (ML) and natural language processing (NLP) to analyze large datasets. It uncovers patterns, trends, and insights that help businesses make data-driven decisions. It's a meeting point of AI, big data, and analytics.
Important Elements
Machine Learning Algorithms: AI analytics heavily relies on ML algorithms for data analysis and prediction modeling.
Big Data Processing: AI analytics can manage and process massive amounts of data efficiently and accurately.
Predictive Analytics: AI analytics allows for predictive modeling, enabling businesses to forecast future trends.
Real-time Analysis: With AI analytics, businesses can analyze data in real-time, aiding quick decision-making.
Automated Insights: AI analytics tools can automatically generate insights, saving time and resources.
B. The Role of AI Analytics in Businesses
AI analytics plays an integral role in businesses by providing insights into customer behavior, enhancing marketing strategies, optimizing operations, and predicting trends. It empowers businesses to leverage their data effectively.
Important Elements
Customer Behavior Analysis: AI analytics helps businesses understand their customers better and personalize their experiences.
Optimized Marketing: With AI analytics, businesses can enhance their marketing strategies and improve ROI.
Operational Efficiency: AI analytics can streamline business operations and increase productivity.
Risk Mitigation: By predicting trends and analyzing patterns, AI analytics helps businesses mitigate risks.
Strategic Decision Making: Data-driven insights from AI analytics aid businesses in making strategic decisions.
C. The Impact of Budget Constraints on AI Adoption
Budget constraints can limit the adoption of AI in businesses, especially small and medium-sized ones. While the potential benefits of AI analytics are undeniable, the initial investment for infrastructure and talent can be substantial.
Important Elements
High Initial Investment: The cost of setting up AI infrastructure can be a hurdle for businesses with budget constraints.
Talent Acquisition: Finding professionals skilled in AI and analytics can be challenging and costly.
Maintenance Costs: The ongoing costs of maintaining and upgrading AI systems can be significant.
Cost-Benefit Analysis: Businesses must balance the cost of implementing AI with the potential benefits.
Budget AI Tools: The rise of budget AI tools is democratizing AI, making it accessible to businesses of all sizes.
III. Determining Your AI Analytics Needs
A. Identifying Your Data Analysis Requirements
The first step in selecting the right AI analytics tool is to identify your business's data analysis requirements. You need to understand the volume, velocity, and variety of data your business generates and what insights you wish to extract from it.
Important Elements
Volume: Depending on the size of your business, the volume of data generated can vary. Large companies might require more robust AI analytics tools.
Velocity: This refers to the speed at which data is generated and processed. If your business deals with real-time data, you need a tool that can keep up.
Variety: Different businesses generate different types of data. Ensure your tool can handle structured and unstructured data alike.
Insight Extraction: Identify what insights you need from your data to align your choice with your business goals.
Compliance: Choose a tool that complies with data privacy and protection laws pertinent to your business.
B. Understanding the Impact of Your Business Size and Type
Your business size and type greatly influence your AI analytics tool choice. A startup might not need as sophisticated a tool as a multinational corporation, and a manufacturing company will have different data analysis requirements than a digital marketing agency.
Important Elements
Startups vs Corporations: While startups might opt for budget-friendly, simpler AI tools, large corporations may require complex, feature-rich AI analytics tools.
Industry-Specific Needs: A healthcare provider might require an AI tool that specializes in patient data analysis, while a retail business might need a tool adept at analyzing customer behavior and sales data.
Cloud vs On-Premise: Depending on your business size, you might choose a cloud-based AI analytics tool for its scalability, or an on-premise solution for better control over your data.
Software Integration: Your AI analytics tool should seamlessly integrate with your existing software infrastructure.
Security: Larger businesses might be more susceptible to cyber attacks, making security a paramount feature.
C. Importance of Scalability in Choosing a Tool
Scalability is key when choosing an AI analytics tool. As your business grows, your data analysis needs will evolve. The tool you choose should be able to scale and adapt to your changing needs.
Important Elements
Growing Data Volume: As your business expands, so will your data. Choose a tool that can handle increasing data volumes.
Evolving Data Complexity: Over time, you may need to analyze more complex data sets. Your tool should be capable of handling this.
Increasing User Base: As more team members start using the AI analytics tool, it should maintain performance without compromise.
Feature Upgrades: The tool should offer regular feature upgrades to meet your evolving business needs.
Cost Effectiveness: As the tool scales, the cost should remain effective for your business.
IV. The Intersection of Cost and Quality
A. How Price Influences Quality in AI Analytics
Navigating the terrain of AI analytics tools can be daunting, especially when you have to strike a balance between cost and quality. The correlation between price and quality is a crucial aspect to consider in your AI tool selection journey.
Tips and Tricks with Intriguing Facts
Invest Wisely: Higher-priced tools often come with advanced features like deep learning capabilities and improved language understanding. However, don't presume that the most expensive tool is automatically the best fit for your needs. Always relate the tool's features back to your specific business requirements.
Fact: AI tools like GPT-4 and LaMDA are recognized for their advanced LLM model and language understanding features, but their cost might not suit all businesses.
Trial Runs: Many AI tools offer free trials or demo versions. Make use of these to assess whether the tool's quality matches its price tag.
Fact: HubSpot CRM, a popular AI tool, provides a free version that businesses can use before deciding on a paid subscription.
Open-Source vs Commercial: Open-source tools can be a cost-effective alternative to commercial AI analytics tools. They often have a supportive community for problem-solving and enhancements.
Fact: AI tools like Jasper and Socratic, although not as robust as some commercial alternatives, provide ample capabilities for startups or small businesses.
Scalability = Long Term Savings: Consider whether the tool can grow with your business. A slightly more expensive tool that is scalable might save costs in the long run.
Fact: AI platforms such as Perplexity AI and Google Bard emphasize scalability, a feature that can prove to be cost-effective in the long term.
Security and Compliance: Compliance with data security regulations is a must, regardless of the tool's price. Data breaches can result in hefty fines and damage to your brand's reputation.
Fact: AI tools that prioritize data security might cost more but can prevent potential losses due to non-compliance.
B. Possible Trade-offs When Opting for Budget Tools
When opting for budget AI analytics tools, understanding the potential trade-offs is crucial. These may include limited features, less comprehensive customer support, and slower innovation rates.
Tips and Tricks with Intriguing Facts
Limited Features: Budget tools might not have the broad feature set that more expensive counterparts offer. Ensure that it meets your essential requirements.
Fact: AI tools like Ink, while affordable, might not offer features like advanced NLP capabilities seen in more expensive tools.
Customer Support: Budget tools might offer less comprehensive customer support. Research the tool's customer support reputation before committing.
Fact: Some affordable AI tools, like Ryter, still prioritize excellent customer service, proving that cost and quality customer support aren't always directly correlated.
Slower Innovation: Lower-cost tools might not update or innovate as quickly as their pricier counterparts, which could impact your tool's longevity.
Fact: More expensive AI tools, such as those offered by OpenAI and Microsoft, frequently update and innovate due to larger development budgets.
Integration Challenges: Budget tools may not integrate as smoothly with other software or platforms. Assess their integration capabilities during the trial period.
Fact: AI tools like HubSpot software are known for their seamless integration capabilities across various platforms, which might not be as robust in some budget options.
Scalability Limitations: Cheaper tools might not be able to handle increasing data volumes or evolving business requirements.
Fact: Even though tools like Chatsonic and YouChat might be budget-friendly, they might lack the capacity blocks and scalability offered by more expensive tools.
V. Top 5 Budget AI Analytics Tools: An Overview
Venturing into the world of AI analytics doesn't necessarily mean breaking the bank. Several budget-friendly tools on the market offer impressive functionality. The Top Budget AI Analytics Tools o 2024:
Let's look at five such tools that are currently trending:
Google Analytics: A free tool with an option for a premium version, Google Analytics offers an abundance of features that help businesses understand customer behavior.
OpenRefine: This open-source tool is ideal for data cleaning and transformation, offering both basic and advanced features.
RapidMiner: It's a data science platform that offers a free version of their software, providing comprehensive data mining and machine learning capabilities.
Tableau Public: A free version of Tableau, this tool allows for data visualization and sharing across platforms.
KNIME: An open-source, free analytics platform that provides over 2000 modules for data analysis.
VI. Deep Dive: Google Analytics
A. Detailed Features
Google Analytics offers an array of features tailored to both small businesses and large enterprises.
These features include:
Audience Reports: Provides insights into the demographics and interests of your website visitors.
Behavior Reports: Tracks how visitors interact with your website.
Conversion Tracking: Monitors key metrics like sales, downloads, and sign-ups.
Real-Time Reporting: Shows what's happening on your site at any given moment.
Mobile App Analytics: Tracks app engagements and conversions.
B. Pricing Structure
Google Analytics operates on a freemium model. The free version offers robust capabilities for small to mid-sized businesses. For larger enterprises requiring more advanced features, Google Analytics 360 is available for a monthly fee.
C. A Case Study of Successful Usage
Online retail giant, Zappos, utilized Google Analytics to gain insights into their website's shopping behavior, enabling them to increase their conversion rate significantly.
VII. Deep Dive: OpenRefine
A. Detailed Features
OpenRefine is known for its strong data cleaning capabilities and flexible transformation commands.
Key features include:
Data Cleaning: Allows users to clean and transform data easily.
Data Transformation: Facilitates transforming data across multiple cells simultaneously.
Reconciliation: Matches local data with existing online databases.
Web Scraping: Can extract data from HTML pages.
Extensions: Additional functionalities can be added via extensions.
B. Pricing Structure
Being an open-source tool, OpenRefine is free to use.
C. A Case Study of Successful Usage
The British Library used OpenRefine to clean and standardize their digital datasets, improving the accessibility and usability of their online catalog.
In the world of AI analytics, there is a tool for every budget. Choosing the right one requires understanding your specific needs, budget constraints, and the level of complexity you can handle. With a thorough investigation, you can find a tool that offers high value for your investment.
VIII. Deep Dive: RapidMiner
A. Detailed Features
RapidMiner is lauded for its robust data mining and predictive analytics capabilities.
The key features include:
Data Preprocessing: Allows users to clean, transform, and reduce data complexity.
Machine Learning: Offers over 1500 machine learning models and algorithms.
Model Validation: Facilitates the testing of machine learning models for reliability.
Data Visualization: Presents data insights in an easily digestible graphical format.
Deployment: Assists in operationalizing predictive models.
B. Pricing Structure
RapidMiner offers a free version with limited features. Their paid versions, RapidMiner Studio and RapidMiner Go, provide more advanced capabilities at different price points.
C. A Case Study of Successful Usage
Samsung used RapidMiner for predictive maintenance of their production facilities, helping to increase their overall operational efficiency.
IX. Deep Dive: Tableau Public
A. Detailed Features
Tableau Public is a free tool known for its data visualization capabilities.
The main features include:
Data Blending: Integrates data from various sources into a unified view.
Real-Time Collaboration: Allows multiple users to work on the same visualization.
Data Notification: Sends alerts when the data behind a visualization changes.
Sharing: Enables users to share visualizations via blogs and social media.
Mobile-Ready Dashboards: Allows for interactive dashboard viewing on mobile devices.
B. Pricing Structure
Tableau Public is free for use. Tableau offers more advanced tools at different price points for business needs, including Tableau Desktop and Tableau Server.
C. A Case Study of Successful Usage
The City of Boston used Tableau Public to create an interactive map of city services, making it easier for residents to access information.
X. Deep Dive: KNIME
A. Detailed Features
KNIME is an open-source data analytics tool, offering
A variety of features:
Data Preprocessing: Facilitates cleaning and reducing data complexity.
Data Mining: Provides tools to analyze large datasets.
Machine Learning: Supports various machine learning algorithms.
Integration: Works seamlessly with numerous data formats and systems.
Visualization: Presents complex data in a clear, visual format.
B. Pricing Structure
KNIME is free to use as it is an open-source platform. For enterprise-level needs, they offer a server version at a certain price.
C. A Case Study of Successful Usage
Lufthansa, the German airline, leveraged KNIME to optimize their customer service operations, leading to significant improvements in customer satisfaction scores.
Choosing the right AI analytics tool involves understanding your business needs and budget constraints. The right tool can turn a deluge of data into actionable insights, improving your decision-making process and positively impacting your business outcomes.
XI. Comparison of the Top 5 Tools
A. Comparison of Features
Here's how our top five AI analytics tools stack up in terms of features:
Data Preprocessing: All tools have a focus on data preprocessing, with Alteryx and RapidMiner taking the lead due to their extensive range of features.
Machine Learning Capabilities: RapidMiner and KNIME outshine others with a comprehensive suite of machine learning models and algorithms.
Data Visualization: Tableau Public and Google Analytics excel in data visualization, transforming complex data into easy-to-understand graphics.
Integration: All five tools offer various levels of integration, with Alteryx and Google Analytics integrating seamlessly with a vast array of other platforms.
Collaboration: Tableau Public and Google Analytics stand out for their robust collaboration features, enabling real-time data analysis and sharing.
B. Comparison of Pricing Structures
Differences in pricing structures can greatly affect which tool is best suited for your budget:
Free Versions: Google Analytics and Tableau Public offer robust features in their free versions, providing significant value without any monetary investment.
Paid Options: Alteryx, RapidMiner, and KNIME offer paid versions with more advanced features, with Alteryx being the most expensive.
Subscription vs One-Time Fee: While most tools offer a subscription-based pricing model, KNIME provides a one-time payment option for its server version.
Enterprise Pricing: All tools offer enterprise pricing, with cost varying depending on the size and specific needs of the business.
Cost-effectiveness: Google Analytics and Tableau Public provide the most cost-effective solutions, especially for small businesses or individual users.
C. Determining the Best Value for Money
Identifying the best value for money involves balancing cost and features:
User-Friendliness: Google Analytics and Tableau Public are user-friendly, offering intuitive interfaces that lessen the learning curve.
Feature-Rich: RapidMiner and KNIME offer a comprehensive suite of features, providing substantial value for more technically advanced users.
Scalability: Alteryx and Google Analytics offer great scalability, making them suitable for businesses with growth plans.
Return on Investment (ROI): Alteryx, despite being more expensive, can deliver a higher ROI for large businesses due to its extensive features and capabilities.
Free vs Paid: Google Analytics and Tableau Public's free versions offer excellent value for money, while the advanced features in the paid versions of RapidMiner and Alteryx provide value for more complex needs.
XII. Other Noteworthy Budget AI Analytics Tools
In addition to our top 5 AI analytics tools, there are several other options worth considering for those on a budget. These solutions have a range of functionalities and pricing models, ensuring there's something for every business size, type, and budget.
A. Quick Overview of Other Options
Microsoft Power BI: Microsoft's entry into the data analytics sphere offers a robust set of tools for data visualization and business intelligence. Power BI provides seamless integration with other Microsoft products, which can be a significant advantage for businesses heavily invested in the Microsoft ecosystem. It also offers a competitive pricing structure, starting with a limited free version, with more extensive capabilities available in the paid version.
Zoho Analytics: A comprehensive solution for businesses seeking advanced AI analytics capabilities without a hefty price tag. Zoho Analytics boasts impressive machine learning capabilities, smart data alerts, and intuitive data visualization tools. Its pricing model is flexible, with a free version available for small-scale needs and a range of subscription options for larger businesses.
IBM Watson Analytics: An AI-powered data analytics and visualization service that caters to businesses of all sizes. Watson Analytics offers predictive analytics, data mining, and trend analysis, along with user-friendly data exploration. However, it leans toward the more expensive side, making it suitable for businesses with a larger budget.
DataRobot: DataRobot leverages automated machine learning to empower users to build and deploy highly accurate predictive models. This solution focuses on ease of use and accessibility, allowing non-experts to harness the power of machine learning. DataRobot's pricing is upon request, but reviews suggest it provides excellent value for the cost.
Qlik Sense: Known for its associative analytics engine and sophisticated AI, Qlik Sense provides interactive data visualization and exploration. It allows users to create personalized reports and dashboards with drag-and-drop simplicity. Qlik Sense has a tiered pricing model, with a free version available for basic needs and paid options offering more advanced capabilities.
A comparative table showcasing the features, pros, and cons of each of the budget-friendly AI analytics tools.
Tool | Features | Pros | Cons |
Microsoft Power BI | Data visualization, business intelligence, integration with Microsoft products | Seamless integration with Microsoft ecosystem, Competitive pricing, Extensive capabilities | Limited features in free version |
Zoho Analytics | Advanced AI, smart data alerts, intuitive data visualization tools | Flexible pricing, Impressive machine learning capabilities | Higher learning curve for beginners |
IBM Watson Analytics | Predictive analytics, data mining, trend analysis | User-friendly data exploration, Advanced capabilities | More expensive than other tools |
DataRobot | Automated machine learning, predictive models | User-friendly, Ideal for non-experts | Pricing not transparent |
Qlik Sense | Associative analytics engine, sophisticated AI, interactive data visualization | Personalized reports and dashboards, Drag-and-drop simplicity | Limited capabilities in the free version |
XIII. Adopting AI Analytics: The Implementation Process
A. Steps to Adopting an AI Analytics Tool
Identifying Needs: Clearly understand and outline what you need from an AI analytics tool. Are you looking for predictive analytics, data mining, or visualization features?
Market Research: Conduct thorough market research to find tools that fit your needs. Look for tools that are reliable, have good customer reviews, and match your budget constraints.
Vendor Interaction: Reach out to the vendors, request demos, ask for detailed features, and discuss pricing models.
Implementation: Once you've decided on a tool, begin the implementation process. This might involve integrating the tool with your current systems, importing data, and setting up relevant workflows.
Employee Training: Organize extensive training sessions for your team members to make sure they're comfortable using the new tool.
B. Common Pitfalls to Avoid During Implementation
Lack of Clear Goals: Without a clear understanding of what you want to achieve with AI analytics, your implementation might lack direction and prove less effective.
Ignoring Data Quality: Even the best AI analytics tool can't provide accurate insights if the data fed into it is of low quality or irrelevant.
Overlooking Change Management: Implementing AI analytics tools isn't just about technology. It involves changing workflows and possibly even organizational culture. Overlooking this aspect can lead to resistance among team members.
Neglecting Ongoing Training: Regularly update training programs to account for updates or changes in the AI analytics tool.
Failure to Monitor: Monitor the tool's effectiveness continuously and be prepared to make necessary adjustments to ensure it's providing the expected value.
Here's a detailed table with a breakdown of each step of adoption, potential challenges that may arise, and solutions to overcome these challenges.
Steps of Adoption | Challenges | Solutions |
Identifying Needs | Lack of understanding of technical jargon; difficulty defining requirements | Conduct thorough needs analysis; leverage expert help if needed |
Market Research | Overwhelming number of tools in the market; understanding which features are necessary | Look for tools with high user ratings; Consult with tool vendors; Request demos |
Vendor Interaction | Time-consuming; possibility of not getting complete information | Prepare a list of questions beforehand; request detailed feature lists |
Implementation | Compatibility issues with existing systems; data migration challenges | Hire experienced implementation teams; Ensure data quality before migration |
Employee Training | Resistance to change; learning curve | Effective change management; regular, comprehensive training sessions |
XIV. The Future of Budget AI Analytics
The world of AI analytics is constantly evolving, and with the right blend of foresight and strategy, businesses can ensure they're ahead of the curve. Let's delve into the up-and-coming trends to monitor in the AI analytics market and explore their potential implications for budget tools.
A. Trends to Watch in the AI Analytics Market
Automated Machine Learning (AutoML): AutoML platforms are streamlining the way organizations develop, refine, and apply machine learning models. These platforms will likely lower the barriers to entry for AI analytics.
Explainable AI (XAI): As AI becomes more complex, the need for transparency increases. XAI, which offers insights into how AI models make decisions, will become critical.
Real-time Analytics: The demand for real-time insights is growing, pushing AI analytics tools to develop capabilities for instantaneous data processing and analysis.
Privacy-focused AI: With increased scrutiny on data privacy, we're likely to see AI analytics tools that are specifically designed to respect and protect user data.
Integration with other tech trends: Expect to see AI analytics tools integrated with other technology trends, like IoT, blockchain, and edge computing.
B. How These Trends Can Impact the Future of Budget Tools
More accessible AI: With AutoML, AI analytics will be more accessible, which could drive down costs and make budget tools even more powerful.
Transparency leads to trust: XAI could make budget AI tools more trustworthy as users will understand how data is being analyzed and used.
Real-time insights: Real-time analytics can give budget tools an edge, making them highly appealing for businesses needing immediate insights.
Privacy is a priority: Budget tools that prioritize privacy could stand out in the market, offering added value to their users.
Expansive integrations: The more integrations a budget tool offers with other technologies, the more value it will provide to businesses, potentially disrupting the current market dynamics.
Let's consider a potential future scenario for each of the trends and a corresponding strategy to leverage the trend to boost efficiency and discover epic insights.
Trends | Future Scenarios | Proven Tactics | Efficiency Boosters | Epic Discoveries |
AutoML | The dominance of AutoML platforms simplifies machine learning applications | Train your team to use AutoML tools for predictive modeling | Automating the creation of ML models frees up resources for strategy and implementation | Insights from complex data sets become accessible without needing a dedicated data science team |
XAI | AI becomes more complex, but XAI keeps it transparent and understandable | Utilize XAI tools to fully understand AI decision-making processes | Increases trust in AI analytics and accelerates decision-making | Decision-makers gain a better understanding of how AI comes to certain conclusions |
Real-time Analytics | Real-time data becomes the norm, necessitating instant analytics | Implement real-time analytics to provide up-to-date insights | Enables immediate decision-making and response to market changes | Uncover insights as they occur, allowing for immediate action |
Privacy-focused AI | Data privacy laws become stricter | Invest in privacy-preserving analytics tools to ensure compliance | Reduces the risk of privacy breaches and the associated penalties | Discovery of a balance between data utility and data privacy |
Integration with other tech trends | AI analytics tools become a hub of various integrated technologies | Identify which tech trends (IoT, Blockchain) are most beneficial for your business and seek tools that integrate them | Allows for a more streamlined workflow with diverse technologies | Breakthrough integrations could lead to unprecedented business insights |
XV. Conclusion
Navigating the world of budget AI analytics tools doesn't need to be complex. By understanding the intersection of cost and quality, and by comparing the top tools on the market, you can make an informed decision that best suits your needs. It's essential to remember that implementation is as critical as selection; a successful adoption involves a comprehensive plan and a keen awareness of common pitfalls. Looking forward, trends such as AutoML, XAI, and others are set to revolutionize the AI analytics market further, making this the right time to embrace AI analytics.
Key Takeaways
Cost vs. Quality: An investment in AI analytics is a delicate balance between cost and quality. While budget tools can offer excellent value, ensure you're not compromising crucial features for your business.
Choosing the Right Tool: Compare the features, pricing structure, and case studies of successful usage of the top tools in the market. Consider your business needs and select a tool that aligns with them.
Successful Adoption: Have a detailed plan for implementing the AI analytics tool into your business. Avoid common pitfalls, such as not having the right skillset in your team or underestimating the integration time.
Future Trends: Stay abreast of the latest trends in AI analytics like AutoML, XAI, real-time analytics, privacy-focused AI, and integration with other tech trends. These trends will likely shape the future of AI analytics tools.
Final Thoughts: The future of budget AI analytics is promising. With the right tool and a comprehensive implementation strategy, businesses of all sizes can leverage AI analytics to gain insights, enhance efficiency, and stay competitive.
These key takeaways provide a roadmap for navigating the world of AI analytics tools. As with any significant decision, careful research and consideration are vital. But the potential benefits are immense, making it a worthy endeavor for businesses aiming to leverage the power of AI in their analytics.
XVI. FAQs:
What are the essential features to look for in a budget AI analytics tool?
When evaluating budget AI analytics tools, consider factors such as ease of use, scalability, data visualization capabilities, predictive analytics features, real-time analytics, and privacy measures. The tool should align with your business goals and have a proven record of success.
How can a small business benefit from AI analytics?
How does the implementation of an AI analytics tool impact daily business operations?
What are some common challenges when adopting AI analytics, and how can they be overcome?
What are the top 5 budget AI analytics tools?
What are some upcoming trends in AI analytics?
How can I determine the best value for money when choosing an AI analytics tool?
What are some noteworthy budget AI analytics tools other than the top 5?
What is the process of adopting an AI analytics tool?
How can future trends impact budget AI analytics tools?
Comments