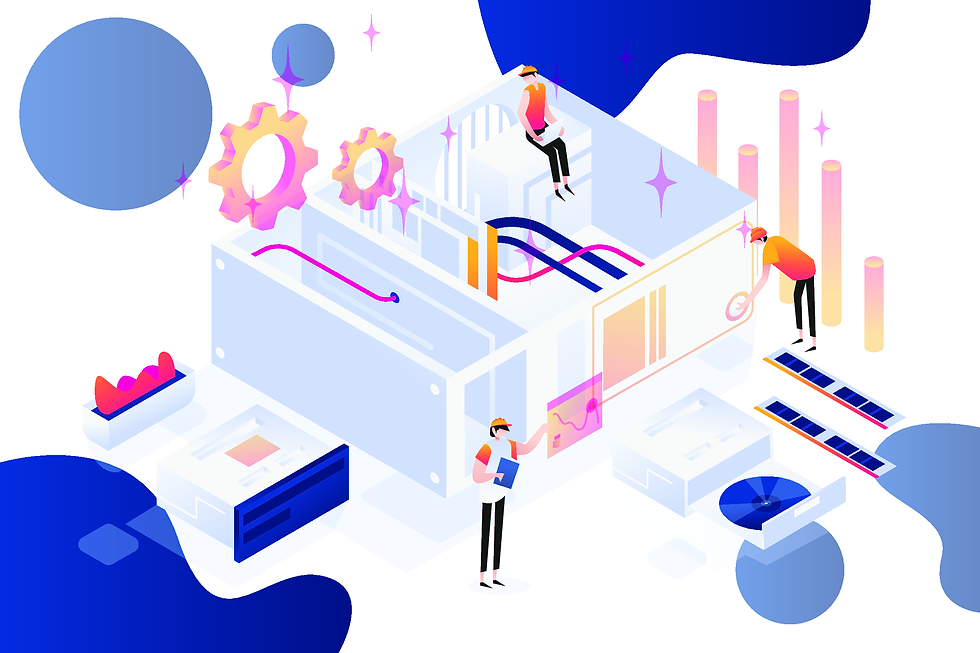
Setting the Stage for AutoML
The Evolution of AutoML: A Brief Look at its History
AutoML: What it is and Why it Matters
Key Features of an AutoML Tool
AutoML vs Traditional Machine Learning: A Comparative Analysis
The Role of AI in AutoML's Growth
The Impact of Data Privacy Regulations on AutoML Tools
Criteria for Selection
A Glimpse into the Top Contenders
Overview and Key Features
Strengths and Limitations
User Experiences: Real-world Scenarios
Overview and Key Features
Strengths and Limitations
User Experiences: Real-world Scenarios
Overview and Key Features
Strengths and Limitations
User Experiences: Real-world Scenarios
Overview and Key Features
Strengths and Limitations
User Experiences: Real-world Scenarios
Overview and Key Features
Strengths and Limitations
User Experiences: Real-world Scenarios
Overview of New Entrants
Impact and Potential: A Foreseeable Future
Understanding Your Needs
Key Considerations: Pricing, Ease-of-use, Customizability
Current Industry Predictions
Emerging Trends to Watch
Summary of Insights
Closing Thoughts on the Future of AutoML
Navigating the Future of AutoML: Tool Reviews and User Experiences 2024
1. Introduction to AutoML in 2024
Setting the Stage for AutoML
In 2024, we stand on the cusp of an automated revolution. Automation, already integral in many sectors, has now penetrated the realms of machine learning, bringing forth a wave of AutoML tools. AutoML, or Automated Machine Learning, is designed to make the complex world of machine learning accessible to non-experts and improve efficiency of experts. Picture a world where machines take over the tedious task of developing machine learning models - that's the stage AutoML is setting.
The Evolution of AutoML: A Brief Look at its History
AutoML didn't just spring up overnight. Its roots lie in the early days of artificial intelligence and machine learning, where researchers envisioned systems that could learn and improve over time. Remember, the idea of teaching machines to learn like humans do has been around since the 1950s, but it's only in the last decade that we've seen significant strides in this direction. The rise of cloud computing, big data, and advanced algorithms, coupled with the growing demand for AI expertise, have all contributed to the birth and growth of AutoML.
2. Understanding AutoML: The Core Concepts
AutoML: What it is and Why it Matters
Navigating the Future of AutoML: Tool Reviews and User Experiences 2024: AutoML, or Automated Machine Learning, is a method of automating the process of applying machine learning to real-world problems. In simpler terms, it's a way to create machine learning models without needing to be a machine learning expert. It matters because it opens the door to ML and AI applications for businesses that lack ML expertise. Plus, it drastically reduces the time taken to develop and deploy models, enabling quicker, data-driven decisions.
Key Features of an AutoML Tool
An ideal AutoML tool typically includes features like automated data pre-processing, model selection, hyperparameter tuning, and model evaluation. It might also provide a graphical user interface for ease of use and feature importance to identify which variables are contributing most to the model's predictions. Imagine you're baking a cake and you have an assistant that chooses the right ingredients (data pre-processing), picks the perfect baking time (model selection), sets the ideal oven temperature (hyperparameter tuning), and checks if the cake is cooked perfectly (model evaluation). Sounds helpful, right? That's what an AutoML tool essentially does in the machine learning world.
AutoML vs Traditional Machine Learning: A Comparative Analysis
At a glance, the main difference between traditional machine learning and AutoML lies in their levels of automation and user-friendliness. In traditional machine learning, ML engineers require a deep understanding of different algorithms, and it can be a time-consuming process to manually tune parameters and test different models. Conversely, AutoML automates many of these steps, making it possible to develop models more quickly and efficiently. It's like comparing manual driving, where you control every move, to autonomous driving, where the car does most of the work for you.
3. Trends Shaping AutoML in 2024
The Role of AI in AutoML's Growth
Artificial Intelligence (AI) is the secret sauce that's fueling the growth of AutoML. AI technologies, such as deep learning and reinforcement learning, allow AutoML tools to become smarter and more efficient. Let's break it down.
Deep Learning: Imagine your brain with its intricate network of neurons. Now imagine a machine trying to mimic that. That's deep learning. AutoML tools use deep learning to extract and learn complex patterns from large datasets.
Reinforcement Learning: Think of a child learning to walk. Every step taken is a learning experience. Similarly, AutoML tools use reinforcement learning to try different algorithms and settings, learning over time which ones work best.
The Impact of Data Privacy Regulations on AutoML Tools
In the era of GDPR and CCPA, data privacy regulations have a profound impact on AutoML tools. These tools need access to data to function effectively. However, with stricter privacy laws, the challenge is to maintain the performance of these tools without compromising user privacy.
Anonymization: AutoML tools are increasingly using techniques to anonymize data, ensuring that the identities behind the data points remain undisclosed.
On-Premise Solutions: To adhere to data sovereignty laws, some AutoML tools are providing on-premise solutions, enabling data to remain within the company's infrastructure.
Differential Privacy: This is a method that adds 'noise' to the data, making it difficult to identify individual data points, while still allowing overall trends to be observed. It's like blending into a crowd — you're still there, but no one can point you out.
Table: Tips and Tricks for Navigating Data Privacy with AutoML
Tips | Description |
Understand the Data Privacy Laws | Be well versed with the privacy laws applicable to your region and industry. |
Use Privacy-Preserving AutoML Tools | Opt for AutoML tools that prioritize data privacy and provide relevant features. |
Regularly Audit Your Data Practices | Regular audits can help ensure your data practices remain compliant with privacy laws. |
AI's Future Forecast: Comparing the Best AutoML Tools with Traditional ML Tools in 2024
4. Unveiling the Top AutoML Tools of 2024
Criteria for Selection
When it comes to selecting an AutoML tool, there's no one-size-fits-all. The right tool for you depends on your specific needs. However, here are some common criteria to consider:
Ease of Use: Does the tool require extensive machine learning knowledge, or is it designed for beginners?
Customizability: Can you tweak the models, or is it a black-box solution?
Data Privacy: Does the tool provide features to protect data privacy?
Scalability: Can the tool handle your data as it grows over time?
Pricing: Does the tool fit within your budget constraints?
Table: Pointers to Look for in an AutoML Tool
Pointers | Description |
Integration with existing systems | Ensure the tool can integrate smoothly with your existing tech stack. |
Customer Support | Look for tools that provide strong customer support, especially if you're new to machine learning. |
Community and Resources | A vibrant community and rich resources can be a great help in your AutoML journey. |
Speed | Consider the speed of the tool — how quickly can it produce accurate models? |
Trial or Free Version | Before committing, try the tool out to see if it fits your requirements and skill level. |
A Glimpse into the Top Contenders
When it comes to the AutoML arena, several big players are making a splash in 2024. Tools from tech giants like Google Cloud AutoML and IBM AutoAI are continuing to dominate the landscape with their robust offerings. At the same time, newer entrants like DataRobot and H2O.ai's AutoML are impressing users with their innovative features and user-friendly interfaces. Stay tuned as we dive deeper into these tools in the coming sections.
5. AutoML Tool Review: Google AutoML
Overview and Key Features
Google's AutoML is a collection of tools built to help folks who aren't experts in machine learning. Some standout tools in this collection include:
Cloud AutoML Vision: This tool allows users to train custom machine learning models to classify images.
Cloud AutoML Natural Language: This tool uncovers the hidden structure and meaning within text data.
Cloud AutoML Tables: This tool enables teams to automatically construct and deploy cutting-edge machine learning models.
Strengths and Limitations
Strengths:
Its interface is designed for ease of use, inviting non-experts to get involved in machine learning.
Google's Cloud platform provides robust computing capabilities and scalability.
Google AutoML offers a rich variety of features for both structured and unstructured data.
Limitations:
Its pricing model might be steep for small businesses or individual users.
It offers less customization compared to manual machine learning approaches.
User Experiences: Real-world Scenarios
Online Retailer: An online store used Google AutoML Vision to create a custom image classification system, improving the way they organize product images.
Journalism Outlet: A news organization employed Google AutoML Natural Language to automate the categorization of articles, which helped streamline their content management.
Insurance Firm: An insurance company applied Google AutoML Tables to predict claim amounts more accurately, leading to improved risk management.
6. AutoML Tool Review: DataRobot
Overview and Key Features
DataRobot aims to make data science accessible to all. Its automated machine learning platform takes care of everything from data preparation to model deployment. Some of its prominent features include:
Automated feature engineering: Simplifies the process of creating new features from the data.
Model validation and selection: Automates the process of choosing the best model.
Automated model documentation: Generates the documentation for each model automatically.
Strengths and Limitations
Strengths:
Wide variety of machine learning algorithms to choose from.
Integrates well with other data platforms.
Manages missing data effectively.
Limitations:
Pricing information is not readily available, which could deter some users.
Less suitable for handling unstructured data, such as images or text.
User Experiences: Real-world Scenarios
Retail Business: A retail store used DataRobot for sales forecasting, helping them manage their stock more efficiently and avoid overstocking.
Healthcare Provider: A healthcare provider employed DataRobot to predict patient readmission rates, allowing them to provide proactive care.
Marketing Agency: A marketing firm used DataRobot to predict the success of various ad campaigns, allowing them to allocate their advertising budget more effectively.
7. AutoML Tool Review: H2O.ai's AutoML
Overview and Key Features
H2O.ai's AutoML platform strives for speed, scale, and simplicity. Its main goal is to allow non-experts to work on complex machine learning tasks. Key features include:
Automated feature engineering: This helps to generate new, relevant features from the data automatically.
Model selection: The platform can automatically choose the best model based on the data and problem.
Explainability: H2O.ai places great emphasis on model transparency and interpretability.
Strengths and Limitations
Strengths:
H2O.ai's AutoML excels in handling tabular data and offers robust support for regression and classification tasks.
Its platform is open-source and easily integrates with a variety of other data platforms.
The ability to explain model behavior is a huge plus for sectors requiring model transparency.
Limitations:
It may not be as beginner-friendly as other platforms.
H2O.ai does not handle unstructured data types, such as images or text, as well as some competitors.
User Experiences: Real-world Scenarios
Finance Firm: A finance company used H2O.ai to predict loan defaults accurately, which helped mitigate risk and inform lending decisions.
Retail Company: A retail firm employed H2O.ai for demand forecasting, improving their inventory management and reducing waste.
Healthcare Organization: A healthcare provider used H2O.ai to predict patient outcomes, improving patient care and hospital efficiency.
8. AutoML Tool Review: IBM AutoAI
Overview and Key Features
IBM AutoAI is a part of IBM's Cloud Pak for Data, designed to automate many of the time-consuming aspects of a data scientist's work. Key features include:
Automatic data preparation: It prepares data for machine learning automatically.
Model selection and hyperparameter optimization: This helps choose the best model and fine-tune it for your data.
Model deployment: Deploy models directly to the IBM Cloud with a few clicks.
~ Strengths and Limitations
Strengths:
It's built to integrate seamlessly with IBM's extensive suite of data and AI tools.
It provides robust support for a wide range of machine learning tasks and data types.
IBM AutoAI includes functionality for automatic feature engineering.
Limitations:
The platform might be less accessible to users not already using IBM's data and cloud services.
Some users might find its interface less intuitive compared to competitors.
User Experiences: Real-world Scenarios
Banking Institution: A bank used IBM AutoAI to predict fraudulent transactions, leading to significant reductions in fraud-related losses.
Marketing Company: A marketing agency used IBM AutoAI to optimize ad spend across multiple channels, resulting in increased ROI.
Logistics Company: A logistics company employed IBM AutoAI to predict delivery times, improving customer satisfaction and operational efficiency.
9. AutoML Tool Review: Microsoft Azure's AutoML
Overview and Key Features
Microsoft Azure's AutoML service aims to make machine learning more accessible to non-experts while still providing powerful tools for professionals. Key features include:
Automated machine learning: Azure AutoML automatically iterates over machine learning algorithms and hyperparameters to find the best model.
Model interpretability: Azure offers insights into how the model makes decisions.
Integration: Azure AutoML integrates well with other Azure services.
Strengths and Limitations
Strengths:
Microsoft Azure provides a user-friendly interface along with the powerful backend.
It offers a rich array of machine learning tasks, including text analytics and image classification.
Azure's global infrastructure provides scalability and robustness.
Limitations:
Like IBM AutoAI, it may be less accessible to users not already invested in Microsoft's cloud and data services.
The pricing model might be expensive for small businesses and individual users.
User Experiences: Real-world Scenarios
E-commerce Platform: An e-commerce company used Azure AutoML to build a recommendation system, leading to an increase in average order value.
Manufacturing Company: A manufacturer used Azure AutoML for predictive maintenance, reducing downtime and improving efficiency.
Healthcare Institution: A healthcare institution employed Azure AutoML to analyze medical images, improving diagnostic accuracy.
10. Emerging Players in the AutoML Landscape
In 2024, we're witnessing a new wave of innovators in the AutoML landscape. These emerging players are shaking up the industry with their innovative approaches and disruptive technologies.
Overview of New Entrants
Here are a few that stand out:
Snorkel AI: This startup focuses on transforming the way organizations label their datasets, a crucial and time-consuming part of the ML process.
Trifacta: Trifacta is pioneering data wrangling automation, making the data pre-processing step more efficient.
Zetaops: Zetaops is an innovative startup that uses AutoML to streamline complex operational processes.
Remember, these are just a few of the many newcomers making waves in the AutoML sector.
Impact and Potential: A Foreseeable Future
These emerging players hold immense potential. They're making AutoML more accessible and efficient, promising a future where businesses can harness the power of ML without needing a team of data scientists.
11. Choosing the Right AutoML Tool: Factors to Consider
Understanding Your Needs
Choosing an AutoML tool isn't a one-size-fits-all scenario. The best tool depends on your unique needs. Do you need a tool with powerful data preprocessing capabilities, or is model explainability your main concern? Identify your needs first.
Key Considerations: Pricing, Ease-of-use, Customizability
Here are some factors you should consider:
Pricing: Ensure the tool fits within your budget constraints.
Ease-of-use: Choose a tool that's user-friendly, especially if your team isn't made up of ML experts.
Customizability: Some tools offer more flexibility and customization options than others.
Integration: Ensure it integrates well with your existing tech stack.
Support and Community: Tools with robust user communities and support can be beneficial.
12. The Future of AutoML: Predictions and Trends
Current Industry Predictions
Looking ahead, the AutoML market is set to flourish. Experts predict that:
AutoML will democratize machine learning, making it more accessible to non-experts.
The use of AutoML in business operations and decision-making will become the norm.
Transparency and explainability will become key focus areas in AutoML development.
Emerging Trends to Watch
Keep an eye out for these trends:
Greater Emphasis on Data Privacy: With increasing privacy regulations, AutoML tools will need to prioritize data privacy.
Rise of Meta-learning: This involves teaching AutoML algorithms to improve themselves based on past experience.
Increased Model Explainability: As AutoML gains adoption, demand for transparency and understanding of how models make decisions will increase.
13. Conclusion: The State of AutoML in 2024
Navigating the future of AutoML unveils a transformative landscape. We've journeyed through the world of AutoML, assessing the top tools including Google AutoML, DataRobot, H2O.ai's AutoML, IBM AutoAI, and Microsoft Azure's AutoML, each presenting unique strengths and real-world application scenarios.
Emerging players, such as Snorkel AI, Trifacta, and Zetaops, hint at a promising future with innovative AutoML applications. However, the selection of the right AutoML tool should be based on individual needs, pricing, ease-of-use, and customizability.
Looking ahead, the industry predicts trends such as meta-learning, increased model explainability, and a focus on data privacy. In essence, AutoML in 2024 is set to democratize machine learning, making it accessible beyond data scientists and experts, and fostering data-driven decision making in businesses.
Here are the key takeaways:
AutoML is democratizing the field of machine learning, making it accessible to a larger audience.
Top AutoML tools offer unique strengths and cater to different user needs.
Emerging players are innovating and expanding the possibilities in the AutoML landscape.
Selecting the right AutoML tool involves understanding individual needs, cost, and usability factors.
Future trends in AutoML include meta-learning, enhanced model explainability, and a focus on data privacy.
As we move forward, critical questions remain: Which AutoML tool is best for beginners? How are data privacy concerns addressed? Can AutoML replace data scientists? Understanding these will be crucial in making informed decisions in the evolving world of AutoML.
FAQ’s:
What is AutoML and why is it crucial in 2024?
AutoML or Automated Machine Learning is an approach that automates the process of applying machine learning to real-world problems. In 2024, it is crucial as it democratizes machine learning, simplifies complex processes, and enhances productivity.
What is the best AutoML tool for beginners?
How have AutoML tools evolved over the years?
How do AutoML tools handle data privacy concerns?
Can AutoML replace data scientists?
What factors should be considered when choosing an AutoML tool?
How much does a typical AutoML tool cost?
Who are the emerging players in the AutoML landscape?
What are some common pitfalls to avoid when using AutoML tools?
What are the predicted trends for AutoML's future?
Comentários