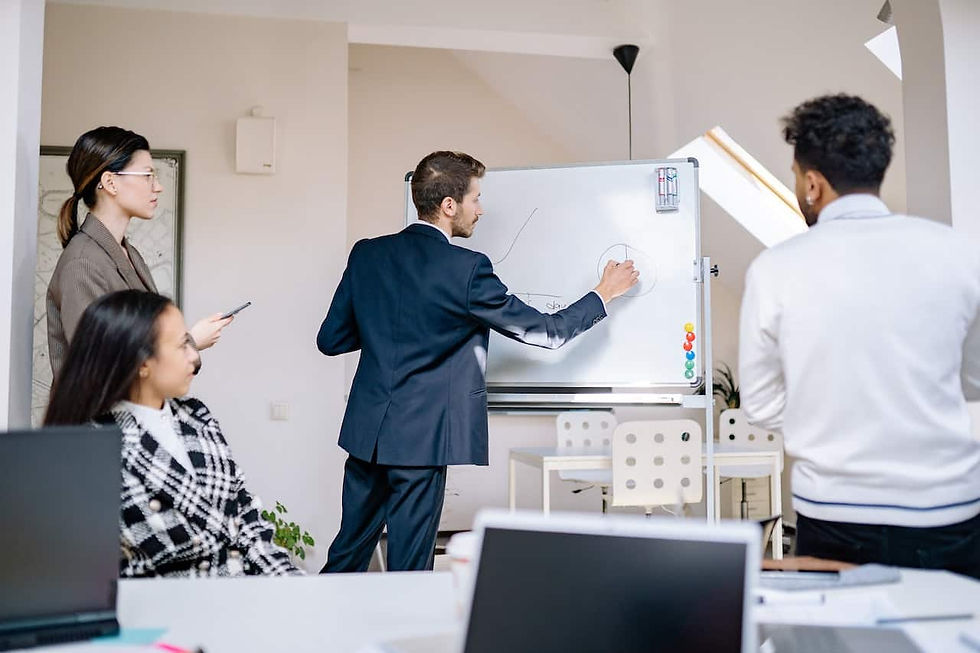
I. Introduction
A. Brief Overview of AI in Trading
B. The Rise and Significance of AI Algorithms
C. Purpose and Scope of the Article
A. Basic Concepts of AI Algorithms in Trading
B. Advantages of Implementing AI in Trading
C. Limitations and Risks of AI Algorithms in Trading
A. AI in Trading: The Early Years
B. Developments and Trends Through the 2020s
C. The Current State of AI in Trading (2024)
A. AI-powered Hedge Funds
B. Algorithmic Trading in the Stock Market
C. Predictive Analytics in Commodity Trading
A. AI and Machine Learning Algorithms
B. Reinforcement Learning in Trading
C. Evolutionary Algorithms and Trading
A. Predictive Modelling and Data Mining
B. AI-based Sentiment Analysis for Market Trends
C. Deep Reinforcement Learning for High-Frequency Trading
D. Quantum Computing in Algorithmic Trading
A. Regulatory Landscape for AI in Trading
B. Ethical Considerations and Fairness in AI Trading
C. Mitigating Bias and Ensuring Transparency in AI Trading
A. Essential Skills and Knowledge
B. Understanding the Role of AI in Trading Strategy
C. Adoption and Adaptation: Embracing AI in Trading
A. Predictions for AI in Trading (2024-2030)
B. Impact of Emerging Technologies on AI Trading
C. The Role of AI in Sustainable and Responsible Trading
X. Conclusion
A. Recap of Key Points
B. The Transformative Potential of AI in Trading
Innovative ideas for implementing AI algorithms in trading 2024
I. Introduction
Artificial Intelligence (AI), a term once confined to the realm of science fiction, has steadily become a critical component in today's financial industry, radically reshaping the traditional mechanisms of trading. With the relentless pursuit of efficiency, precision, and profitability, the marriage of AI and trading has spawned an exciting epoch of financial innovation, with Algorithmic Trading being one of its flagship advancements. Innovative ideas for implementing AI algorithms in trading 2024:
Brief Overview of AI in Trading: AI has remarkably revolutionized trading by enabling high-speed, data-driven, and automated decisions. Its application ranges from automating mundane tasks to predicting market trends, shaping and redefining the landscape of financial trading.
The Rise and Significance of AI Algorithms: The realm of algorithmic trading has been catapulted into prominence in recent years, with AI algorithms efficiently parsing colossal datasets to glean valuable insights for quantitative trading. They aid in mitigating risks, enhancing accuracy, and maximizing profits in the ever-volatile stock market.
Purpose and Scope of the Article: This article aims to demystify the intersection of AI and trading, providing a comprehensive exploration of innovative AI implementation ideas in trading as of 2024. This journey shall illuminate the myriad ways AI algorithms can augment the financial industry and equip the reader with insights into this rapidly evolving domain.
II. Understanding AI Algorithms in Trading
The essence of successful trading lies in interpreting intricate patterns, making timely decisions, and skillfully managing risks — attributes at which AI algorithms excel.
Basic Concepts of AI Algorithms in Trading: AI algorithms refer to intricate computational procedures, which harness machine learning techniques to analyze financial markets data, generate trading signals, and execute trades autonomously. Algo Trading leverages these algorithms to make trading decisions, manage orders, and optimize trading speed, contributing to more effective investment strategies.
Advantages of Implementing AI in Trading: AI brings numerous benefits to the financial trading arena. AI algorithms can process vast quantities of data far beyond human capacity, identifying market patterns and trends for more informed investment decisions. Furthermore, AI allows for algorithmic trading, mitigating emotional and human errors, and it enables 24/7 trading due to its automated nature. AI also promotes quantitative trading, where complex mathematical models help devise strategies for maximum profitability.
Limitations and Risks of AI Algorithms in Trading: As with any technology, AI in trading comes with its caveats. The risk of overfitting, where an AI model overly adapts to historical data and performs poorly on new data, is a common concern. There's also the risk of flash crashes due to high-frequency, AI-powered trades. Lastly, AI systems are only as good as the data they're trained on, making them susceptible to garbage-in, garbage-out scenarios.
III. Historical Evolution of AI in Trading
The integration of Artificial Intelligence in the financial industry has been an evolution rather than a revolution, with each advancement acting as a stepping stone towards the current state of algorithmic trading.
AI in Trading: The Early Years:
The genesis of AI in trading dates back to the late 20th century, where rudimentary AI systems were utilized for simple tasks such as identifying basic trends and executing straightforward trades. The landscape of financial markets was very different back then, with AI serving as a novelty rather than a necessity.
Main ideas:
Emergence of AI in the late 20th century
Basic trend identification and trade execution
AI as a novelty
Developments and Trends Through the 2020s:
The advent of machine learning and advanced computational power in the 2020s ushered in an era of sophisticated AI trading systems. Machine learning algorithms for predictive analytics in trading became increasingly prevalent, and quantitative trading gained traction. These advancements paved the way for AI-powered investment strategies that harnessed big data to optimize trading decisions.
Main ideas:
Advent of machine learning
Use of predictive analytics in trading
Emergence of quantitative trading
The Current State of AI in Trading (2024):
Fast forward to 2024, and AI is not just a part of the financial industry – it's at its very core. With AI's capability to analyze vast amounts of financial data, the transition to algorithmic trading has been revolutionary, enabling efficient and profitable trades even in volatile stock market conditions.
Main ideas:
AI at the core of the financial industry
Transition to algorithmic trading
AI's ability to operate in volatile market conditions
IV. Case Studies: Successful Implementation of AI in Trading
AI's impact on trading is best understood through real-world examples, where its successful implementation has transformed trading strategies and results.
AI-powered Hedge Funds:
Hedge funds like Renaissance Technologies and Two Sigma have harnessed AI's predictive analytics to drive superior returns. These funds use AI for algorithmic trading, making millions of trades per day based on patterns and predictions derived from colossal data sets.
Main ideas:
Renaissance Technologies and Two Sigma as examples
Use of predictive analytics in trading
High-volume, AI-based trades
Algorithmic Trading in the Stock Market:
Firms such as Citadel and Virtu Financial have used AI algorithms to dominate the high-frequency trading landscape in the stock market. These firms employ quantitative trading strategies to execute trades at lightning speed, leveraging minute price discrepancies for profit.
Main ideas:
Citadel and Virtu Financial as examples
Dominance in high-frequency trading
Use of quantitative trading strategies
Predictive Analytics in Commodity Trading:
Companies like Cargill and Vitol use AI's predictive analytics capabilities to forecast commodity price movements, optimizing their buying and selling strategies. With AI's data analysis, these firms can predict market trends, ensuring they stay ahead of the curve.
Main ideas:
Cargill and Vitol as examples
Use of predictive analytics for commodity trading
AI's role in predicting market trends
V. Exploring Innovative AI Algorithms in Trading
As we enter a new era of algorithmic trading, it's crucial to understand the innovative AI algorithms that are shaping the financial industry. This includes:
AI and Machine Learning Algorithms:
These algorithms form the foundation of modern trading systems. They utilize AI and machine learning to analyze vast quantities of financial data, learning patterns, and making data-driven decisions.
Main ideas:
Critical role of AI and machine learning in trading
Ability to process large data sets
Learning and improving from data patterns
Reinforcement Learning in Trading:
A type of machine learning, reinforcement learning involves teaching AI systems through a process of rewards and punishments. This has shown to be incredibly effective in the dynamic world of trading.
Main ideas:
Application of reinforcement learning in trading
Training AI through rewards and punishments
Adapting and optimizing investment strategies
Evolutionary Algorithms and Trading:
Taking inspiration from biological evolution, these algorithms use mechanisms such as mutation and crossover to discover and refine trading strategies. They represent a promising future direction for algorithmic trading.
Main ideas:
Use of evolutionary algorithms in trading
Mechanisms like mutation and crossover for strategy refinement
The promising future of these algorithms in trading
AI's Future Forecast: Surf the AI Wave: AI-Powered Analytics Industry Innovations in 2024
VI. Future Trading: Innovative Ideas for Implementing AI
As we look to the future, several innovative ideas are poised to redefine AI implementation in trading:
Predictive Modelling and Data Mining:
These techniques will be critical in extracting valuable insights from vast datasets, facilitating informed investment decisions.
Main ideas:
Role of predictive modelling and data mining in future trading
Uncovering valuable insights from large datasets
Facilitating informed investment decisions
AI-based Sentiment Analysis for Market Trends:
Using AI for sentiment analysis can provide an edge in predicting market trends. This process involves analyzing online data to gauge market sentiment, providing unique insights into market movement.
Main ideas:
Use of AI for sentiment analysis
Predicting market trends based on online data analysis
Unique insights into market movements
Deep Reinforcement Learning for High-Frequency Trading:
A blend of deep learning and reinforcement learning, deep reinforcement learning promises to revolutionize high-frequency trading by enabling faster and more accurate trade executions.
Main ideas:
Use of deep reinforcement learning in high-frequency trading
Faster and more accurate trade executions
The future potential of this technology in trading
Quantum Computing in Algorithmic Trading:
Quantum computing holds vast potential for algorithmic trading. Its capabilities could lead to unprecedented speeds and efficiencies in trading systems.
Main ideas:
The potential of quantum computing in trading
Speed and efficiency improvements in trading systems
The future implications of quantum computing in algorithmic trading
VII. Regulatory Challenges and Ethical Considerations
The use of AI in trading has raised the bar of trading efficiency, but it also brings along a unique set of regulatory challenges and ethical considerations. Here, we'll dissect these issues and offer key insights.
A. Regulatory Landscape for AI in Trading
AI brings speed and precision to trading, but it's not immune to regulatory oversight.
Understanding the regulatory framework can be a daunting task, but it's crucial for the lawful operation of AI in trading.
Traders need to remain aware of the local, national, and international regulations regarding AI use in trading.
B. Ethical Considerations and Fairness in AI Trading
Ethical use of AI in trading means ensuring it doesn't exacerbate inequalities or reinforce biases.
Fairness in AI trading involves equal access to AI resources and unbiased algorithmic decision-making.
Traders should work towards making AI trading tools accessible and preventing biases in data or AI models.
C. Mitigating Bias and Ensuring Transparency in AI Trading
Mitigating bias in AI models is essential to maintain fair markets and traders' trust.
Transparency in AI-driven decisions is key to accountability and confidence in trading operations.
Regular audits and transparent disclosure of AI decision-making processes can help mitigate bias and ensure transparency.
VIII. Preparing for AI-Driven Trading: A Guide for Traders
AI-driven trading is the future. It's time traders prepare to steer their trading operations in this AI-centric direction.
A. Essential Skills and Knowledge
Traders need to master AI concepts, data analysis, and machine learning.
Constant learning is key to staying abreast of advancements in AI trading technology.
Interpreting AI outputs effectively and integrating them into trading strategies can help make trading operations more efficient and profitable.
B. Understanding the Role of AI in Trading Strategy
AI can automate complex trading tasks, analyze market trends, and predict future movements.
Proper integration of AI in trading strategy can enhance decision-making and increase profit margins.
Traders should strive to understand and leverage the capabilities of AI to improve their trading strategies.
C. Adoption and Adaptation: Embracing AI in Trading
Embracing AI in trading is a progressive journey involving learning, adaptation, and continual evolution.
Adapting to AI-driven trading involves adjusting trading strategies, learning new skills, and complying with emerging regulations.
Successful integration of AI into trading can lead to enhanced trading outcomes, but traders must also consider the ethical and regulatory aspects.
Plan for Embracing AI in Trading
Objectives
Master AI Concepts
Engage in continual learning through courses, seminars, etc.
Increase in knowledge and skills related to AI.
Integrate AI in Trading Strategy
Understand AI capabilities and leverage them in trading strategies
Improved decision-making and increased profit margins.
Ensure Ethical AI Trading
Regularly audit AI models for bias and ensure transparency in decision-making processes
Decrease in biased outcomes and increased transparency.
Comply with Regulations
Stay updated on regulatory developments and adapt trading operations accordingly
Compliance with local, national, and international regulations.
IX. Looking Ahead: The Future of AI in Trading
As we traverse through the digital era, the role of AI in trading is becoming more pronounced and pivotal. This new trading horizon promises thrilling possibilities but also imposes new challenges. Let's explore what lies ahead.
A. Predictions for AI in Trading (2024-2030)
Predicting the future can be tricky, but some trends indicate exciting developments in AI trading. Between 2024 and 2030, we anticipate:
Enhanced AI algorithms that offer higher accuracy in market predictions.
Increased adoption of AI in trading across the globe.
Evolution of AI-powered tools that help traders make more informed decisions.
B. Impact of Emerging Technologies on AI Trading
Emerging technologies continue to augment AI's capabilities in trading. Some of these technologies include:
Quantum Computing: Could drastically speed up complex calculations, leading to faster trading decisions.
Blockchain: Offers a secure environment for AI-based trading, minimizing the risk of fraudulent activities.
Internet of Things (IoT): Provides real-time data collection from various sources, enriching AI's data analysis and prediction capabilities.
C. The Role of AI in Sustainable and Responsible Trading
Sustainable and responsible trading is no longer optional; it's an imperative. AI can play a crucial role in this transformation:
AI can identify sustainable investment opportunities by analyzing ESG (Environmental, Social, and Governance) data.
AI-powered tools can help traders understand the impact of their decisions on the environment and society.
Through AI, traders can align their strategies with global sustainability goals.
Features, Benefits, and Drawbacks of AI in Trading
High-speed Data Processing
AI can process vast amounts of data in milliseconds, providing real-time insights.
Too much data can lead to overfitting, where the AI model might fail to generalize its predictions.
Predictive AnalyticsAI uses historical data to predict future market trends, helping traders make informed decisions.
Predictive analytics is not infallible and can sometimes result in inaccurate predictions.
Autonomous Decision-making
AI can execute trades autonomously, enhancing efficiency and reducing human error.
Autonomous trading can lead to market vulnerability if AI systems malfunction or are manipulated.
Sustainability AnalysisAI can analyze ESG data to identify sustainable investments.
The quality of sustainability analysis heavily depends on the quality and integrity of ESG data.
The integration of AI into trading is a colossal shift, marking a new era in the financial world. By understanding and addressing the challenges and by leveraging the immense possibilities, we can ensure a future where AI acts as a valuable ally to traders. It's time to embrace this AI-driven future of trading. Remember, the only constant in technology is change. So, let's keep learning, adapting, and innovating!
Comments