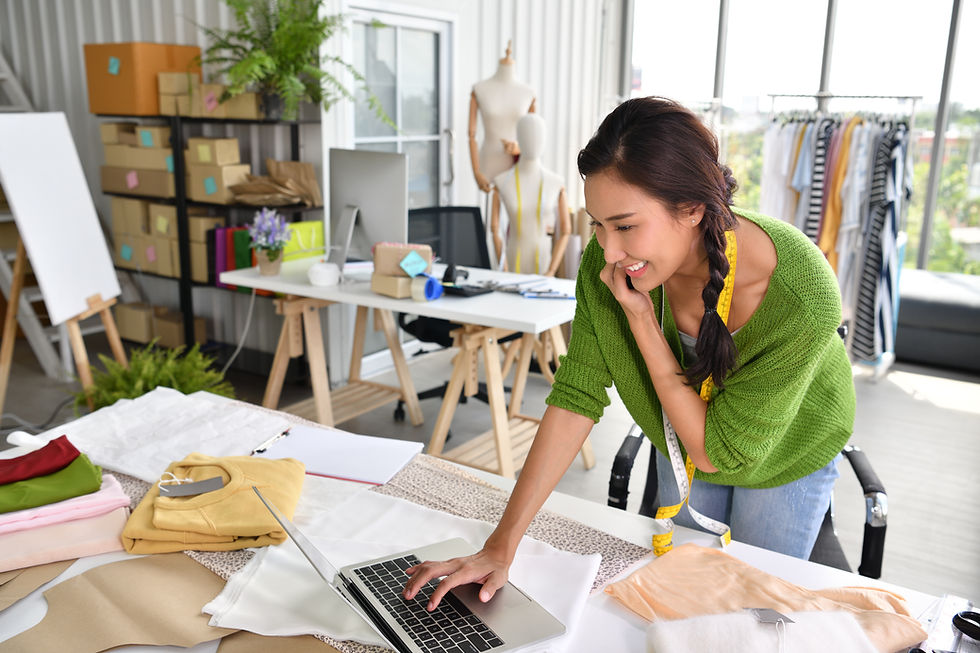
1. Introduction
1.1 Overview of the eCommerce Landscape in 2024
1.2 The Rise of Predictive Analytics in eCommerce
2.1 Definition and Explanation of Predictive Analytics
2.2 How Predictive Analytics Works in the eCommerce Context
2.3 Real-world Examples of Predictive Analytics in eCommerce
3.1 Explanation and Key Characteristics of Conventional eCommerce Methods
3.2 Examples of Conventional Methods Still in Use
4.1 Operational Efficiency: Speed, Cost, and Scale
4.2 Customer Insights: Depth and Breadth of Information
4.3 Adaptability: Reaction to Market Trends and Changes
4.4 Future-proofing: The Sustainability of Each Method
5.1 Improved Customer Experience and Personalization
5.2 Enhanced Supply Chain Management
5.3 Increased Sales through Precise Targeting
5.4 Risks Mitigation and Proactive Problem Solving
6.1 Data Privacy and Security Concerns
6.2 The Need for Skilled Data Analysts
6.3 The Potential for Misinterpretation of Data
7.1 Simplicity and Familiarity of Conventional Methods
7.2 Predictability and Control over Outcomes
7.3 Limitations and Costs Associated with Adopting New Technologies
8. Conclusion
8.1 The Future of Predictive Analytics and Conventional Methods in eCommerce
8.2 Final Thoughts on Choosing the Right Approach for Your eCommerce Business
eCommerce Battle: Much awaited Predictive Analytics vs Conventional Methods of 2024
1. Introduction
The eCommerce landscape is continuously evolving, shaped by the confluence of new technologies, consumer behaviors, and market dynamics. In the year 2024, it has become increasingly evident that predictive analytics and artificial intelligence (AI) tools are emerging as crucial determinants for success in the highly competitive arena of online retail. This blog post will dive into the fascinating world of predictive analytics in eCommerce, its comparison with conventional methods, and the potential implications for the industry's future.
1.1 Overview of the eCommerce Landscape in 2024
The year 2024 has witnessed a continued explosion in electronic commerce as a myriad of businesses, ranging from small online shops to multinational digital retail giants, have leveraged internet retail strategies to reach a global customer base. The use of AI solutions, especially in predictive analytics, has dramatically transformed the eCommerce landscape. Personalized shopping experiences, powered by AI software, are now the norm rather than the exception, enhancing customer journeys and increasing sales and conversions. Online store owners have employed AI-powered eCommerce tools like AI chatbots for seamless customer interactions, machine learning algorithms for optimized pricing policies, and risk and fraud management systems to ensure secure transactions. AI-driven content creation tools have revolutionized content marketing strategies, leading to increased customer engagement and higher conversion rates.
1.2 The Rise of Predictive Analytics in eCommerce
As we delve deeper into the AI technologies shaping 2024's eCommerce landscape, predictive analytics stands out as an influential player. It employs AI software and machine learning tools to analyze historical data, recognize patterns, and make accurate future predictions. The utility of predictive analytics spans across multiple facets of eCommerce:
AI-driven customer behavior analysis provides in-depth insights into individual shopping habits, facilitating personalized marketing strategies.
Predictive analytics coupled with neural networks optimize pricing strategies, taking into account competitor prices and market trends.
The tool aids in supply chain optimization, enabling efficient inventory management and reducing operational costs.
It assists in risk mitigation, identifying potential frauds before they materialize, enhancing the security measures of the online store.
Note: These bullet points are designed to provide a snapshot of key aspects to look out for in the market:
The application of AI tools and predictive analytics in creating personalized shopping experiences and optimizing pricing policies.
The adoption of machine learning algorithms for risk and fraud management.
The increasing importance of AI-driven content creation in enhancing customer engagement and conversion rates.
The continuing relevance of conventional methods in certain areas of eCommerce.
2. What is Predictive Analytics?
Predictive analytics, a subset of advanced analytics, utilizes various statistical techniques, including AI, machine learning algorithms, data mining, and modeling, to analyze current and historical facts and predict future outcomes. The beauty of predictive analytics lies in its ability to use existing data to forecast individualized trends. This predictive foresight provides an edge in strategizing and decision-making processes, paving the way for businesses to capitalize on potential opportunities and mitigate impending risks.
Key Elements of Predictive Analytics | Importance |
Use of historical and current data | Provides a base for predictions |
Utilizes AI and Machine Learning | Enhances prediction accuracy |
Individualized trend forecasting | Facilitates personalization and targeted strategies |
Future-oriented | Allows proactive decision-making and risk mitigation |
2.1 Definition and Explanation of Predictive Analytics
Predictive analytics leverages data, machine learning tools, AI software, and statistical algorithms to identify the likelihood of future outcomes based on historical data. The objective is to go beyond knowing what has happened to provide the best assessment of what will happen in the future. Essentially, predictive analytics provides businesses with a forward-looking perspective, helping them anticipate and prepare for future scenarios. It is like a compass for businesses, guiding them through the vast sea of data towards profitable ventures and away from potential pitfalls.
Main ideas | Implications |
Leveraging data | Harnesses valuable insights from vast amounts of data |
Use of AI and ML tools | Enables more accurate and efficient prediction |
Forward-looking perspective | Aids in proactive decision-making and strategic planning |
Business compass | Guides businesses towards profitable decisions and away from risks |
2.2 How Predictive Analytics Works in the eCommerce Context
In the eCommerce arena, predictive analytics harnesses customer data to optimize various business aspects, such as sales and conversion rates, pricing policies, and customer engagement. For instance, it utilizes machine learning algorithms to analyze customer behavior, enabling personalized marketing strategies and enhancing user experience. It uses AI technology for price optimization, considering competitor prices and market trends. Additionally, predictive analytics aids in fraud detection, offering valuable security measures for online retailers.
Steps in eCommerce Predictive Analytics | Implications |
Collection of customer data | Forms the base for predictive analysis |
Analysis using AI and ML | Unveils patterns and correlations in data |
Personalization of marketing strategies | Enhances user experience and engagement |
Price optimization | Maximizes profitability |
Fraud detection | Enhances security and trustworthiness |
2.3 Real-world Examples of Predictive Analytics in eCommerce
Predictive analytics has manifested in multiple eCommerce applications, transforming online shopping experiences. For instance, Amazon uses predictive analytics for personalized product recommendations, dramatically boosting its sales. Similarly, Netflix uses it for their AI-powered recommendation engine, significantly enhancing user engagement and retention. Zara employs predictive analytics in inventory management, allowing them to maintain an optimal stock level and reduce waste. These real-world examples demonstrate the transformative potential of predictive analytics in enhancing customer experiences, optimizing operations, and maximizing profits in the eCommerce industry.
Real-world Examples | Impacts |
Amazon's personalized recommendations | Enhanced customer experiences and increased sales |
Netflix's AI-powered recommendation engine | Improved user engagement and retention |
Zara's inventory management | Optimized operations and reduced waste |
3. Conventional Methods in eCommerce
Even in the AI-dominated landscape of 2024, conventional methods continue to play a significant role in the eCommerce industry. These methods, based on traditional marketing and sales strategies, serve as the foundational pillars of eCommerce, supporting the sophisticated AI and predictive analytics tools of today.
Key Features of Conventional Methods | Benefits | Potential Drawbacks |
Proven and time-tested strategies | Trusted reliability and predictability | May lack innovation and adaptability |
Simplicity and ease of implementation | Reduced complexity and resource requirements | May fall short in addressing unique, complex scenarios |
Human touch in customer service | Personalized, empathetic customer interactions | May not scale effectively with increasing customer base |
3.1 Explanation and Key Characteristics of Conventional eCommerce Methods
Conventional eCommerce methods are rooted in traditional marketing and sales principles. These methods rely heavily on human judgment, intuition, and past experiences rather than data-driven algorithms. For instance, pricing strategies might be set based on competitors' prices and a business owner's understanding of customer willingness to pay, without involving complex machine learning algorithms. Similarly, customer service might be delivered through human representatives instead of AI chatbots, providing a personalized touch but potentially lacking in scalability. Content creation in conventional methods relies on human creativity and market understanding, devoid of AI-powered content generation tools.
Key Characteristics of Conventional eCommerce Methods | Implications |
Reliance on human judgment and intuition | Adds a human touch but lacks data-driven precision |
Pricing based on competitors and customer willingness | May miss opportunities for optimized pricing |
Human-delivered customer service | Provides personalization but may lack scalability |
Human-driven content creation | Employs creativity but may lack data-driven insights |
3.2 Examples of Conventional Methods Still in Use
Despite the advent of AI and predictive analytics, some conventional methods remain relevant in the eCommerce landscape. For instance, email marketing, although an old-school approach, is still a powerful tool for nurturing leads and driving customer engagement. SEO optimization, another conventional method, continues to be crucial in driving organic traffic to eCommerce sites. Even traditional customer service, with its human touch, remains valuable in building customer relationships and ensuring customer loyalty.
Conventional eCommerce Methods | Applications |
Email Marketing | Nurturing leads, driving customer engagement |
SEO Optimization | Driving organic traffic, increasing site visibility |
Traditional Customer Service | Building customer relationships, ensuring customer loyalty |
4. Comparing Predictive Analytics and Conventional Methods
The eCommerce environment in 2024 calls for an in-depth understanding of the distinct features and trade-offs between predictive analytics and traditional methods. We'll cover four primary areas: operational efficiency, customer insights, market adaptability, and sustainability.
4.1 Operational Efficiency: Speed, Cost, and Scale
When considering operational efficiency, defined by speed, cost, and scalability, the differences between predictive analytics and conventional methods become apparent. The power of AI and machine learning algorithms enables predictive analytics to rapidly process huge volumes of data. On the contrary, traditional methods might have lower initial investment costs but cannot match the speed and scalability offered by predictive analytics.
Predictive Analytics | Conventional Methods |
Quick processing time | Slower processing time |
Higher upfront costs | Lower upfront costs |
Can handle massive datasets | Limited in data processing scale |
Quick generation of actionable insights | More time needed for insights |
High efficiency in data usage | Less efficient data usage |
4.2 Customer Insights: Depth and Breadth of Information
Predictive analytics uses AI and machine learning for deeper, more extensive customer insights, which enables more personalized shopping experiences. Conventional methods, while providing a more empathetic view of the customer, lack the ability to provide similarly comprehensive insights.
Predictive Analytics | Conventional Methods |
Detailed customer profiling | General customer profiling |
Broad range of customer behaviors | Limited range of customer behaviors |
Real-time customer insights | Delayed customer insights |
High personalization potential | Limited personalization potential |
Accurate prediction of customer needs | Less precise prediction of customer needs |
4.3 Adaptability: Reaction to Market Trends and Changes
Predictive analytics shows a high degree of adaptability due to its ability to process real-time data. Conventional methods, while providing a level of stability during market volatility, might not respond as swiftly or accurately to market trends and changes.
Predictive Analytics | Conventional Methods |
Rapid response to market changes | Slower response to market changes |
Real-time market trend analysis | Delayed market trend analysis |
Higher agility in strategy adjustment | Lower agility in strategy adjustment |
Accurate prediction of market trends | Less precise prediction of market trends |
High adaptability to new technologies | Lower adaptability to new technologies |
4.4 Future-proofing: The Sustainability of Each Method
Predictive analytics appears to be the future of eCommerce, but its sustainability is contingent on technological evolution and data accessibility. Conventional methods, though seeming outdated, have a track record of sustainability but need to adapt and integrate with technologies such as AI to stay relevant.
Predictive Analytics | Conventional Methods |
Dependent on future tech advancements | Less dependent on tech advancements |
High potential for integration with new tech | Limited integration with new tech |
More reliant on data availability | Less reliant on data availability |
High potential to evolve with market trends | Steady but slower evolution |
Requires constant update of knowledge and skills | Traditional knowledge and skills often suffice |
5. The Benefits of Predictive Analytics in eCommerce
Predictive analytics in eCommerce has several significant advantages. It allows for advanced customization, enhances supply chain management, increases sales through precise targeting, and helps in risk mitigation and proactive problem solving. With such capabilities, predictive analytics is becoming a powerful AI tool that every eCommerce store owner should consider integrating into their digital retail strategy.
5.1 Improved Customer Experience and Personalization
Predictive analytics provides a detailed understanding of a customer's buying behavior, allowing for a more personalized shopping experience. This individualized approach increases customer engagement and interaction, leading to an improved overall customer journey.
Quick Fact: 80% of consumers are more likely to make a purchase when brands offer personalized experiences.
Benefits of Improved Customer Experience | Examples |
Higher customer retention rates | Amazon's personalized product recommendations |
Increased customer satisfaction | Spotify's Discover Weekly personalized playlist |
Enhanced customer loyalty | Netflix's personalized viewing suggestions |
Improved customer engagement | Sephora's personalized beauty advice |
Higher conversion rates | Stitch Fix's personalized styling service |
5.2 Enhanced Supply Chain Management
Predictive analytics improves supply chain management by predicting demand, ensuring efficient inventory management, and aiding in cost management through optimized resource allocation. This results in more efficient operations and cost savings.
Quick Fact: Companies can reduce supply chain costs by 10-40% by implementing predictive analytics.
Benefits of Enhanced Supply Chain Management | Examples |
Optimized inventory levels | Walmart's predictive analytics for inventory management |
Reduced overstock and stockouts | Coca-Cola's demand forecasting using predictive analytics |
Improved delivery times | Amazon's predictive shipping |
Cost-effective resource allocation | IBM's supply chain optimization using predictive analytics |
Increased overall efficiency | Ford's predictive analytics in supply chain management |
5.3 Increased Sales through Precise Targeting
Predictive analytics helps increase sales by analyzing customer behavior and identifying potential buyers for specific products. This allows for precise targeting, leading to increased conversion rates and revenue generation.
Quick Fact: Businesses that use predictive analytics for customer acquisition reported a 73% higher sales rate.
Benefits of Increased Sales through Precise Targeting | Examples |
Improved targeting of potential buyers | Google's predictive analytics in ad targeting |
Higher conversion rates | Amazon's customer segmentation using predictive analytics |
Increased revenue | Zillow's precise targeting using predictive analytics |
Enhanced customer acquisition | Salesforce's predictive lead scoring |
More efficient marketing spend | American Express's predictive modeling for targeted offers |
5.4 Risks Mitigation and Proactive Problem Solving
Predictive analytics can help eCommerce businesses identify potential risks and tackle problems before they escalate. It can be particularly useful in fraud detection and prevention, price optimization, and customer churn prediction.
Quick Fact: Predictive analytics can help businesses reduce fraud by up to 15%.
Benefits of Risks Mitigation and Proactive Problem Solving | Examples |
Improved fraud detection and prevention | PayPal's fraud detection using predictive analytics |
Efficient price optimization | Uber's dynamic pricing based on predictive analytics |
Early identification of potential problems | Credit card companies' fraud prevention using predictive analytics |
Proactive customer churn prediction | Telecommunication companies' churn prediction using predictive analytics |
Optimized risk management strategies | Insurance companies' risk assessment using predictive analytics |
6. Challenges of Implementing Predictive Analytics
While predictive analytics brings numerous benefits to the eCommerce landscape, it is not without its challenges. These can range from data privacy concerns to the need for skilled data analysts, and the potential misinterpretation of data. Nevertheless, these challenges can be navigated strategically, helping businesses to effectively leverage the full power of predictive analytics.
6.1 Data Privacy and Security Concerns
One of the most significant challenges associated with predictive analytics is maintaining data privacy and security. Protecting customer data while effectively employing predictive analytics methods is a critical concern for eCommerce businesses.
Quick Fact: 70% of organizations believe that they are at risk of a data breach.
Data Privacy and Security Challenges | Proven Tactics | Efficiency Boosters | Epic Discoveries |
Protecting sensitive customer data | Implementing strong encryption methods | Regular security audits | The GDPR has increased global awareness of data privacy |
Complying with privacy laws | Updating privacy policies regularly | Training employees on privacy laws | The introduction of the California Consumer Privacy Act |
Preventing data breaches | Deploying advanced cybersecurity measures | Regular software updates | The rise of blockchain technology for data security |
Balancing data access and privacy | Granting selective access to data | Implementing two-factor authentication | The emergence of privacy by design concept |
Managing third-party risks | Careful vetting of third-party service providers | Regular security assessments of third-party providers | The rise of cyber insurance |
6.2 The Need for Skilled Data Analysts
Predictive analytics requires a team of skilled data analysts. Finding, training, and retaining such professionals can be challenging due to the high demand for these skills in the market.
Quick Fact: Demand for data scientists is projected to grow by 28% by 2026.
Skilled Data Analysts Challenges | Proven Tactics | Efficiency Boosters | Epic Discoveries |
Hiring skilled data analysts | Collaborating with reputed recruitment agencies | Continuous learning and development programs | The rise of data science boot camps |
Retaining data analysts | Offering competitive compensation packages | Maintaining a positive work environment | The significant role of data science in the growth of tech giants |
Training and upskilling analysts | Providing regular professional development opportunities | Utilizing online learning platforms | The emergence of AI-powered training platforms |
Managing the high cost of data science teams | Outsourcing to cost-effective locations | Adopting advanced data analytics tools | The success of remote work in tech industries |
Keeping up with the latest data analytics trends | Encouraging attendance at industry conferences and seminars | Regular team brainstorming sessions | The impact of AI and machine learning on data analysis |
6.3 The Potential for Misinterpretation of Data
The misinterpretation of data can lead to inaccurate predictions, which can harm business decisions. Ensuring that data is correctly interpreted is crucial for successful predictive analytics implementation.
Quick Fact: Only 37% of organizations have been successful in their data-driven initiatives.
Misinterpretation of Data Challenges | Proven Tactics | Efficiency Boosters | Epic Discoveries |
Ensuring accurate data interpretation | Regular data audits | Implementing stringent data quality measures | The importance of data literacy |
Avoiding confirmation bias in data interpretation | Encouraging diversity in data teams | Promoting a data-driven culture | The impact of cognitive biases on data interpretation |
Managing noisy and unstructured data | Utilizing advanced data cleaning tools | Automating data pre-processing | The rise of natural language processing |
Dealing with incomplete or missing data | Adopting advanced data imputation methods | Implementing comprehensive data collection strategies | The effectiveness of multiple imputation |
Overcoming the 'black box' issue in AI predictions | Promoting transparency in AI models | Adopting explainable AI (XAI) | The emergence of XAI in data science |
7. Why Some eCommerce Businesses Still Prefer Conventional Methods
In the era of predictive analytics and AI-driven insights, it may surprise you to find that many eCommerce businesses still hold fast to conventional methods. Reasons range from the simplicity and familiarity of traditional methods to the predictability and control over outcomes, and even the limitations and costs associated with adopting new technologies.
7.1 Simplicity and Familiarity of Conventional Methods
Conventional methods in eCommerce, such as email marketing, search engine optimization, and customer surveys, continue to hold their ground because of their simplicity and familiarity.
Fun Fact: Even in 2024, email marketing boasts an impressive ROI of $42 for every $1 spent!
Reasons for Preference | User Examples | Advancements | Future Developments |
Easy to implement | Small businesses without a dedicated tech team | Development of user-friendly marketing tools | Increasing personalization features in email marketing tools |
Familiarity | Companies in industries with a more traditional customer base | Enhancements in customer surveys | Integrating AI into traditional marketing methods |
Proven success | Established businesses with a loyal customer base | Advances in SEO techniques | More sophisticated data analysis tools for conventional methods |
Less reliance on tech expertise | Businesses in areas with limited tech infrastructure | Evolution of email marketing strategies | Combining conventional and modern methods for optimal results |
Lower learning curve | New entrepreneurs starting their first business | Developments in targeting and segmentation | Growth of online resources for learning traditional methods |
7.2 Predictability and Control over Outcomes
Some businesses prefer conventional methods because they offer more predictability and control. While predictive analytics can offer valuable insights, the results are probabilistic and not always within control.
Fun Fact: 91% of marketers believe that poor data quality leads to wasted marketing efforts.
Reasons for Preference | User Examples | Advancements | Future Developments |
Greater control | Businesses with stringent regulatory environments | Improvements in risk management strategies | Combining analytics with traditional methods for greater control |
Predictability of outcomes | Companies in stable, less dynamic markets | Developments in strategic planning | AI for enhancing predictability in traditional methods |
Lower risk | Businesses with less margin for error, such as healthcare | Advances in quality assurance | Using predictive analytics to lower risks in conventional methods |
Ease of measuring outcomes | SMEs with limited resources for complex data analysis | Enhancements in performance metrics | Development of more sophisticated tracking tools |
Less dependency on data quality | Companies with unreliable data sources | Advances in data cleansing tools | Increasing importance of data quality in all methods |
7.3 Limitations and Costs Associated with Adopting New Technologies
Adopting new technologies like predictive analytics can be challenging due to the costs, the learning curve involved, and the need for skilled personnel.
Fun Fact: Small businesses spend 4.9% of their revenue on technology, placing significant importance on cost-effective solutions.
Reasons for Preference | User Examples | Advancements | Future Developments |
High initial costs | Startups and small businesses with tight budgets | The rise of affordable SaaS solutions | More cost-effective solutions in predictive analytics |
Learning curve | Companies without a dedicated data science team | Developments in user-friendly analytics tools | Increasing simplicity and usability of analytics tools |
Training and hiring costs | Businesses in areas with limited access to skilled personnel | Growth of online training platforms | Rising trend of remote work in tech roles |
Integration challenges | Companies with legacy systems | Improvements in system integration solutions | Development of more compatible analytics tools |
Data privacy concerns | Businesses dealing with sensitive customer data | Advances in data security measures | Increasing emphasis on data privacy in technology development |
8. Conclusion
The rise of predictive analytics and the persistent value of conventional methods present an interesting crossroads for eCommerce businesses. While both methods offer unique advantages, the key lies in understanding how to effectively blend the new with the old. Predictive Analytics vs Conventional Methods of 2024 in eCommerce.
8.1 The Future of Predictive Analytics and Conventional Methods in eCommerce
The eCommerce landscape is rapidly evolving, and so are the tools and methodologies employed. Predictive analytics offers a revolutionary way to anticipate customer behavior, optimize operations, and increase sales. Yet, conventional methods continue to provide proven and reliable results, resonating with the less tech-savvy or risk-averse businesses.
Key takeaways:
Predictive analytics offers vast potential, allowing for enhanced personalization, better supply chain management, and risk mitigation.
Despite their potential, predictive analytics brings challenges, including data privacy concerns and the need for skilled data analysts.
Conventional methods still find favor due to their simplicity, predictability, and control over outcomes.
There are costs and limitations linked with adopting new technologies that businesses need to consider.
The future of eCommerce lies in a balanced integration of predictive analytics and conventional methods, suiting the unique needs and capacities of each business.
8.2 Final Thoughts on Choosing the Right Approach for Your eCommerce Business
In the end, the choice between predictive analytics and conventional methods hinges on the unique needs, capacities, and goals of your eCommerce business. Embracing a new technology shouldn't mean discarding proven strategies that continue to yield results. The key is to combine the strengths of both, crafting a unique strategy that fits your business and market landscape.
Key takeaways:
While predictive analytics provides future-oriented insights and possibilities, it's important to balance it with the reliability and control of conventional methods.
Every eCommerce business should evaluate its capabilities, resources, and market dynamics before deciding its mix of predictive and conventional methods.
Keep exploring and learning. eCommerce is an ever-evolving field, and staying up-to-date with the latest trends, tools, and strategies is vital for success.
Never underestimate the power of data. Whether it's being used in predictive analytics or traditional methods, high-quality, accurate data is crucial.
Lastly, customer satisfaction is paramount. All strategies, whether predictive or conventional, should ultimately focus on enhancing the customer experience.
By effectively blending predictive analytics with conventional methods, eCommerce businesses can leverage the best of both worlds, creating powerful, customer-centric strategies that drive growth and success into the future.
9. Frequently Asked Questions (FAQs)
What are some successful examples of Predictive Analytics in eCommerce?
Several eCommerce businesses have harnessed predictive analytics for success. Amazon, for example, uses predictive algorithms for product recommendations, while Zara employs it for optimizing its supply chain. Other examples include eBay's predictive analytics for targeted advertising and Netflix's use of predictive modeling for personalizing content.
Is Predictive Analytics the end of Conventional Methods in eCommerce?
What are some of the risks associated with Predictive Analytics in eCommerce?
How can small eCommerce businesses begin to implement Predictive Analytics?
What are the main benefits of predictive analytics in eCommerce?
Why do some eCommerce businesses still prefer conventional methods?
How does predictive analytics improve customer experience in eCommerce?
What is the role of a data analyst in predictive analytics?
What are the limitations of adopting predictive analytics in eCommerce?
How is predictive analytics different from conventional methods in eCommerce?
コメント