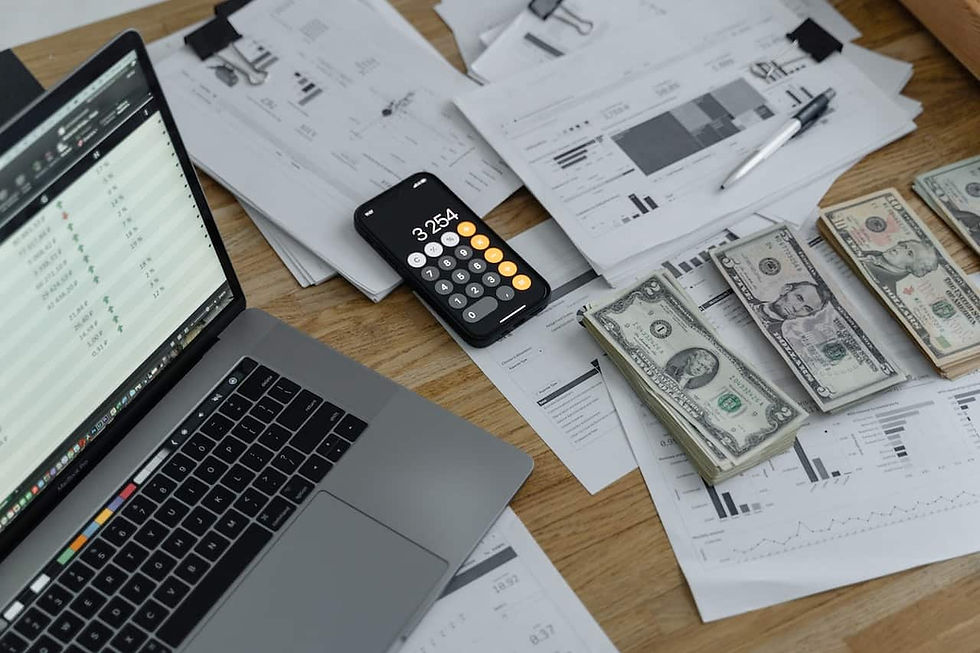
I. Introduction
A. Brief Introduction to Automated Machine Learning (AutoML)
B. The Intersection of AutoML and Finance
C. Why the Financial Sector Needs AutoML in 2024
A. The Evolution of Machine Learning
B. The Mechanisms of AutoML
C. Benefits of Using AutoML in Business Processes
A. The Role of AutoML Tools in Finance
B. AutoML Tools vs Traditional Methods: A Comparative Analysis
A. Google's AutoML
Key Features and Benefits
Applicability in Finance
B. Microsoft's Azure AutoML
Key Features and Benefits
Applicability in Finance
C. DataRobot
Key Features and Benefits
Applicability in Finance
D. H2O.ai’s H2O AutoML
Key Features and Benefits
Applicability in Finance
E. RapidMiner Auto Model
Key Features and Benefits
Applicability in Finance
A. Databricks AutoML
B. Neuraxle's AutoML
C. MindsDB
A. Risk Assessment and Fraud Detection
B. Investment Strategy Formulation
C. Customer Segmentation and Churn Prediction
A. Data Privacy and Security
B. Regulatory Compliance
C. Bias and Fairness in Automated Decision-making
A. Predicted Trends for AutoML in Finance Beyond 2024
B. Preparing for the Future: How Financial Institutions Can Adapt
IX. Conclusion
A. Recap of Key Points
B. Final Thoughts on the Use of AutoML in Finance
The Future of FinTech: A Comprehensive Guide to Recommended AutoML Tools in Finance for 2024
I. Introduction
A. Brief Introduction to Automated Machine Learning (AutoML):
Automated Machine Learning, commonly referred to as AutoML, stands as a significant advancement in the field of Artificial Intelligence (AI). Its primary function is to automate the exhaustive process of applying machine learning to practical problems. The conventional process of machine learning, characterized by intricate tasks like feature selection, model selection, and hyperparameter tuning, requires significant levels of expertise. AutoML simplifies these tasks by automating them, effectively making machine learning more accessible to non-experts while simultaneously enhancing the efficiency of experts. It essentially unlocks the door to Machine Learning Automation, which bears the potential to instigate a revolution across a multitude of sectors, with finance being a prominent one. Recommended Automl tools in finance 2024:
AutoML simplifies complex machine learning processes through automation.
It makes machine learning accessible to non-experts.
AutoML enhances the efficiency of machine learning experts.
It is poised to revolutionize several sectors, including finance.
B. Intersection of AutoML and Finance:
Finance, a field rich in data and numerical information, offers a fertile ground for the applications of AI and Machine Learning. AutoML in finance pertains to the utilization of automated machine learning tools to unravel complex and extensive financial data, which in turn aids in data analysis and decision-making processes. It generates valuable insights into algorithmic trading, quantitative trading, and financial markets, assisting businesses in directing their investment strategies and managing risks. As AutoML tools continue to evolve, becoming smarter and more efficient, their application in finance has grown increasingly significant.
Finance industry is a rich source of data, ideal for AI and ML applications.
AutoML aids in analyzing complex financial data.
It provides insights into trading and financial markets, helping in investment strategies and risk management.
AutoML's role in finance is becoming increasingly significant with its evolution.
C. Why the Financial Sector Needs AutoML in 2024:
In 2024, AutoML stands out as a vital tool for the financial sector. It's immense capacity to analyze enormous datasets and learn from them makes it an invaluable resource in predicting future market trends and investment opportunities with high accuracy. AI-based trading algorithms and automated trading systems, powered by AutoML, play an instrumental role in assessing risks, enhancing financial decision-making quality, and ensuring robust risk management. Furthermore, AutoML can boost the efficiency and speed of high-frequency trading, thereby improving returns on investment. Finally, AutoML is also useful in analyzing customer data, leading to better comprehension of customer behaviors and preferences, and consequently improving customer service and satisfaction.
AutoML enhances predictive analytics, helping in forecasting market trends and investment opportunities.
It assists in risk management through AI-based trading algorithms and automated systems.
AutoML boosts efficiency in high-frequency trading, leading to better investment returns.
It helps improve customer service by analyzing and understanding customer behaviors and preferences.
II. Understanding AutoML
A. The Evolution of Machine Learning
Machine Learning, a subset of Artificial Intelligence, has evolved significantly over the years. It began as a concept grounded in pattern recognition and has expanded to include predictive analytics in trading, financial data analysis, and AI-powered financial modeling. It's now intertwined with our daily lives, from personal assistants like Siri and Alexa to personalized recommendations on platforms like Netflix or Amazon. However, the process of designing machine learning models is intricate and requires expertise, which is where Automated Machine Learning comes into play.
Machine Learning has evolved from simple pattern recognition to complex financial applications.
It has found use in personal assistants and personalized recommendation systems.
Designing machine learning models is complex and needs expertise.
Automated Machine Learning simplifies this process and broadens its accessibility.
B. The Mechanisms of AutoML
AutoML operates by automating the laborious process of building machine learning models. It starts with feature selection, where important variables are chosen from the dataset. Then, it proceeds to model selection where the optimal ML algorithm is picked. Following this, hyperparameters of the chosen model are tuned to improve its performance. Throughout this process, AutoML tracks model performance, ensuring the delivery of an optimal model for prediction or classification tasks. AutoML tools can aid financial industry players in activities like quantitative trading, market trend analysis with AI, portfolio optimization using AI, and AI-based trading algorithms.
AutoML automates the process of feature selection, model selection, and hyperparameter tuning.
It continuously monitors the performance of the model to ensure optimal results.
AutoML aids in various financial industry activities like quantitative trading and market trend analysis.
C. Benefits of Using AutoML in Business Processes
AutoML provides numerous benefits to business processes across sectors. It enables those without extensive knowledge in machine learning to build and implement effective models, thereby democratizing AI. AutoML can optimize resources by significantly reducing the time taken for model development and fine-tuning. It helps businesses to make more informed, data-driven decisions, enhancing areas like risk management with AI, predictive analytics in trading, and AI-driven investment decisions. Furthermore, AutoML supports scalability, allowing models to handle larger datasets and complex computations, aiding in financial decision-making with AI, high-frequency trading with AI, and financial risk assessment with AI.
AutoML democratizes AI by enabling non-experts to build and use ML models.
It optimizes resources by reducing the time for model development and fine-tuning.
AutoML aids in making data-driven decisions in risk management, predictive analytics, and investment.
It supports scalability, handling larger datasets and complex computations.
III. AutoML Tools in Finance: An Overview
When we talk about AutoML tools in finance, we're referring to the utilization of artificial intelligence to automate intricate machine learning tasks. These tools can dramatically streamline various financial operations.
Table outlining the significant features, benefits, and potential drawbacks of AutoML tools:
Features | Benefits | Drawbacks | Compatibility Across Platforms |
Automated Data Pre-processing | Reduces manual data cleaning efforts and errors | Requires high-quality data to begin with | Highly compatible across different platforms |
Model Selection and Hyperparameter Tuning | Boosts accuracy and performance of predictions | May overfit models if not properly managed | Great compatibility, as it's not platform-dependent |
Automated Feature Engineering | Enhances model performance by automatically creating effective features | Could lead to overly complex models | Compatible with many platforms, though some have limitations |
Interpretable AI | Allows for better understanding and trust in model decisions | Interpretability might reduce model performance | Interpretability might reduce model performanceBroad compatibility across platforms, though not all support this |
A. The Role of AutoML Tools in Finance
Finance is a field that’s ripe for AI intervention, and AutoML tools play a central role. They increase efficiency and save time by automating tasks such as data pre-processing, model selection, hyperparameter tuning, and feature engineering. These tools also incorporate interpretable AI, allowing for a deeper understanding and trust in model decisions.
By doing this, AutoML tools help to optimize and streamline operations, resulting in more accurate financial forecasts, risk assessments, algorithmic trading, and fraud detection.
B. AutoML Tools vs Traditional Methods: A Comparative Analysis
In comparing AutoML tools to traditional methods, we see a stark contrast in the way operations are conducted. Traditional methods often rely heavily on manual labor and are time-consuming and prone to error. In contrast, AutoML tools automate and simplify complex tasks, resulting in higher accuracy and efficiency.
However, a significant challenge arises in using AutoML tools. Although they require less human intervention, they still necessitate an understanding of the data and the ability to interpret the results. Therefore, while AutoML tools offer significant advantages, they should be seen as a complement to, rather than a replacement for, financial expertise.
Fundamental building blocks: Comparing the Best AutoML Tools with Traditional ML Tools in 2024
IV. Top Recommended AutoML Tools in Finance 2024
As we look forward to 2024, a host of AutoML tools are ready to revolutionize finance. Here, we highlight five frontrunners: Google's AutoML, Microsoft's Azure AutoML, DataRobot, H2O.ai’s H2O AutoML, and RapidMiner Auto Model.
A. Google's AutoML
Key Features and Benefits
Google's AutoML is a user-friendly tool that provides automated data pre-processing, model selection, hyperparameter tuning, and feature engineering. It excels in its robustness, scalability, and the impressive interpretability of its models.
Applicability in Finance
Google's AutoML shines in tasks like credit risk scoring and financial forecasting. Its ability to process and analyze vast datasets makes it a powerful tool for larger financial institutions.
Tips and Tricks for Google's AutoML
Objective | Action | KPI | Example |
Improve prediction accuracy | Optimize hyperparameters | Increased accuracy of predictions | Optimize hyperparameters for a credit scoring model |
Boost processing efficiency | Automate data pre-processing | Reduced processing time | Automate data cleaning in financial datasets |
Enhance model interpretability | Utilize interpretable AI features | Improved decision-making | Leverage model insights for risk assessment |
Scale operations | Use cloud-based capabilities | Increased operational capacity | Expand processing to larger financial datasets |
Streamline workflow | Integrate with other Google Cloud services | Improved workflow efficiency | Combine Google AutoML with BigQuery for seamless data handling |
B. Microsoft's Azure AutoML
Key Features and Benefits
Azure AutoML, backed by Microsoft's robust cloud infrastructure, stands out with its superior automated machine learning capabilities and deep learning models. It provides a no-code option, making it accessible to non-technical users.
Applicability in Finance
Azure AutoML's powerful deep learning models are well-suited for complex financial applications, such as algorithmic trading, where intricate patterns need to be recognized.
Tips and Tricks for Azure AutoML
Objective | Action | KPI | Example |
Enhance model complexity | Leverage deep learning models | Increased prediction accuracy | Use deep learning for algorithmic trading |
Simplify machine learning tasks | Use the no-code option | Reduced time spent on model building | Employ the no-code feature for risk assessment tasks |
Increase operational capacity | Utilize Microsoft's cloud infrastructure | Ability to process larger datasets | Process vast market data for algorithmic trading |
Improve workflow efficiency | Integrate with other Azure services | Streamlined workflow | Combine Azure AutoML with Azure Databricks for data handling |
Boost data security | Leverage Microsoft's robust security features | Improved data protection | Secure sensitive financial data during processing |
C. DataRobot
Key Features and Benefits
DataRobot is an AutoML tool that's winning the race with its automated best practices and end-to-end platform. It excels at data preparation, feature selection, model building, and deployment. Additionally, it provides excellent interpretability, enabling users to understand the 'why' behind model decisions.
Applicability in Finance
DataRobot can be a game-changer in financial services. Its automated best practices make it ideal for credit scoring, while its end-to-end platform is valuable for tasks such as fraud detection and risk assessment.
Tips and Tricks for DataRobot
Objective | Action | KPI | Example |
Enhance model complexity | Leverage deep learning models | Increased prediction accuracy | Use deep learning for algorithmic trading |
Simplify machine learning tasks | Use the no-code option | Reduced time spent on model building | Employ the no-code feature for risk assessment tasks |
Increase operational capacity | Utilize Microsoft's cloud infrastructure | Ability to process larger datasets | Process vast market data for algorithmic trading |
Improve workflow efficiency | Integrate with other Azure services | Streamlined workflow | Combine Azure AutoML with Azure Databricks for data handling |
Boost data security | Leverage Microsoft's robust security features | Improved data protection | Secure sensitive financial data during processing |
D. H2O.ai’s H2O AutoML
Key Features and Benefits
H2O.ai's H2O AutoML tool comes equipped with a user-friendly interface and powerful ML algorithms. Its capabilities range from data preprocessing to deploying models in production, making it an all-in-one solution.
Applicability in Finance
With its strong capabilities in model building and evaluation, H2O AutoML is perfect for forecasting tasks, anomaly detection, and market segmentation in finance.
Tips and Tricks for H2O AutoML
Objective | Action | KPI | Example |
Enhance prediction accuracy | Use the tool's strong ML algorithms | Increased prediction accuracy | Improve market forecasts |
Boost efficiency | Utilize the all-in-one solution | Streamlined workflow | Unify all ML tasks on one platform |
Improve accessibility | Leverage the user-friendly interface | Reduced time spent learning the tool | Get started quickly with ML tasks |
Increase scalability | Use the tool's scalability features | Ability to handle larger datasets | Analyze extensive market data |
Optimize model deployment | Use the tool's deployment features | Seamless transition to production | Deploy market segmentation models efficiently |
E. RapidMiner Auto Model
Key Features and Benefits
RapidMiner Auto Model is an AutoML tool recognized for its visual workflow designer and its broad suite of ML algorithms. It makes creating complex ML workflows straightforward and intuitive.
Applicability in Finance
RapidMiner shines when it comes to data exploration and understanding complex relationships, making it ideal for tasks such as portfolio management and financial risk modeling.
Tips and Tricks for RapidMiner Auto Model
Objective | Action | KPI | Example |
Simplify ML tasks | Use the visual workflow designer | Reduced time spent building workflows | Create a workflow for financial risk modeling |
Enhance prediction accuracy | Leverage the suite of ML algorithms | Increased prediction accuracy | Improve portfolio management predictions |
Improve data understanding | Utilize the data exploration features | Enhanced decision-making | Explore data for financial risk modeling |
Increase flexibility | Leverage the tool's flexibility features | Ability to handle diverse tasks | Manage a variety of financial tasks |
Boost model validation | Use the tool's validation features | Improved model reliability |
V. Emerging AutoML Tools in 2024 Worth Watching
The future of AutoML in finance looks promising, with a new generation of tools gearing up to revolutionize the way we handle financial data. In this section, we turn our spotlight to three emerging AutoML tools worth keeping an eye on in 2024: Databricks AutoML, Neuraxle's AutoML, and MindsDB.
A. Databricks AutoML
Databricks AutoML, an extension of the Apache Spark-based platform, is showing signs of becoming a significant player in the AutoML sphere. This tool is designed to simplify the creation and tuning of machine learning workflows, thus democratizing AI and opening the doors to a broader user base.
Key Features: It boasts automated feature engineering, model selection, and hyperparameter tuning, along with easy deployment of models in production.
Applicability in Finance: With its robust feature set, Databricks AutoML can streamline a myriad of financial tasks, including risk management, algorithmic trading, and customer segmentation.
B. Neuraxle's AutoML
Neuraxle's AutoML stands out with its emphasis on pipeline design, making the development of complex machine learning workflows a breeze.
Key Features: It offers a flexible, step-by-step approach to ML pipeline construction, including data preprocessing, model building, and performance evaluation.
Applicability in Finance: Neuraxle's pipeline-centric approach can simplify complex financial tasks like multi-asset trading or complex credit scoring models.
C. MindsDB
MindsDB, an open-source AutoML tool, is another rising star, championing transparency and explainability in machine learning.
Key Features: MindsDB stands out with its focus on explainability, empowering users to understand how models arrive at their predictions. It also supports a wide variety of data sources.
Applicability in Finance: The tool's emphasis on explainability makes it an excellent choice for finance, where understanding the 'why' behind predictions is critical—for instance, in credit scoring or fraud detection.
VI. Case Studies: Successful Application of AutoML in Finance
With AutoML’s steady march into the financial sector, several organizations have successfully integrated these tools into their operations, reaping significant benefits. Let's look at a few compelling case studies:
A. Risk Assessment and Fraud Detection
[Insert Image: Visualization of Fraud Detection]
B. Investment Strategy Formulation
Investment strategy formulation can be a complex task. However, with the use of Microsoft Azure AutoML, an asset management company managed to devise effective, data-driven investment strategies. The tool's superior hyperparameter tuning capabilities and seamless integration with the company's existing systems ensured a more efficient and successful investment planning.
C. Customer Segmentation and Churn Prediction
Customer segmentation and churn prediction is another area where AutoML shines. A fintech startup used MindsDB to understand its customer behavior better and predict potential churn. With the tool's focus on explainability, the startup was able to understand the reasoning behind the predictions, enabling it to devise effective retention strategies.
VII. Ethical Considerations and Challenges of AutoML in Finance
While AutoML offers numerous benefits, it also presents new ethical challenges that organizations need to address.
A. Data Privacy and Security
Data is the lifeblood of AutoML, but it's crucial to handle it responsibly. Ensuring data privacy and maintaining security should be a top priority when using these tools. Potential solutions include anonymizing data and implementing robust access control measures.
B. Regulatory Compliance
AutoML tools should be compliant with financial regulations. Non-compliance could lead to penalties and reputational damage. Therefore, tools like Google's AutoML, which offer built-in compliance features, are beneficial.
C. Bias and Fairness in Automated Decision-making
AutoML models can unintentionally perpetuate biases present in the training data, leading to unfair outcomes. To mitigate this, organizations should conduct regular bias audits and consider using tools like H2O.ai’s H2O AutoML that incorporate bias detection.
VI. Case Studies: Successful Application of AutoML in Finance
The infiltration of AutoML into the financial sector has been monumental, with many entities successfully integrating these tools to drive strategic initiatives. Let's delve into several compelling case studies:
A. Risk Assessment and Fraud Detection
AutoML has been a game-changer in risk assessment and fraud detection. A renowned global bank used Databricks AutoML to streamline its risk assessment processes. By employing the tool's automated feature engineering and model selection, the bank managed to improve prediction accuracy, leading to significant cost savings.
Case Example: A global banking titan leveraged Databricks AutoML to enhance its risk assessment procedures. By using the tool's sophisticated feature engineering and model selection capacities, the bank amplified prediction accuracy, leading to substantial fiscal savings.
B. Investment Strategy Formulation
Investment strategy formulation can be a complex task. However, with the use of Microsoft Azure AutoML, an asset management company managed to devise effective, data-driven investment strategies. The tool's superior hyperparameter tuning capabilities and seamless integration with the company's existing systems ensured a more efficient and successful investment planning.
Case Example: An asset management firm utilized Microsoft Azure AutoML to craft intricate, data-led investment strategies. The tool's exceptional hyperparameter tuning abilities and easy integration with existing systems guaranteed efficient and effective investment planning.
C. Customer Segmentation and Churn Prediction
Customer segmentation and churn prediction is another area where AutoML shines. A fintech startup used MindsDB to understand its customer behavior better and predict potential churn. With the tool's focus on explainability, the startup was able to understand the reasoning behind the predictions, enabling it to devise effective retention strategies.
Case Example: A fintech enterprise adopted MindsDB to gain a deeper comprehension of its customer behavior and to forecast potential churn. With the tool's emphasis on explainability, the company could interpret the logic behind the predictions, aiding it in formulating efficient retention plans.
VII. Ethical Considerations and Challenges of AutoML in Finance
While AutoML proffers a myriad of benefits, it simultaneously brings forth a fresh set of ethical quandaries that institutions must confront.
A. Data Privacy and Security
AutoML's reliance on data is profound. However, its responsible handling is paramount. Upholding data privacy and fortifying security should be at the pinnacle when employing these tools. Strategies such as data anonymization and stringent access control implementation could offer potential solutions.
B. Regulatory Compliance
AutoML tools should be compliant with financial regulations. Non-compliance could lead to penalties and reputational damage. Therefore, tools like Google's AutoML, which offer built-in compliance features, are beneficial.
C. Bias and Fairness in Automated Decision-making
AutoML models can unintentionally perpetuate biases present in the training data, leading to unfair outcomes. To mitigate this, organizations should conduct regular bias audits and consider using tools like H2O.ai’s H2O AutoML that incorporate bias detection.
VIII. The Future of AutoML in Finance
The future of finance is inextricably tied to technological advancement. AutoML tools are expected to be significant players in shaping this future, evolving in response to emerging financial trends and requirements.
A. Predicted Trends for AutoML in Finance Beyond 2024
Greater Integration and Adoption: We predict a future where more financial institutions will adopt and integrate AutoML tools into their systems. Their scalability, precision, and versatility make them hard to ignore for any organization serious about staying competitive.
Enhanced Security: As finance is a sensitive sector, we anticipate that AutoML tools will incorporate advanced security features to ward off cyber threats and protect sensitive data.
Regulatory Alignment: With the increased usage of AutoML, we expect to see better alignment with regulatory standards. This means tools that are more compliant with financial regulations out-of-the-box.
Boosted Explainability: The future of AutoML will likely focus on enhancing the interpretability of machine learning models, fostering trust and understanding among decision-makers.
B. Preparing for the Future: How Financial Institutions Can Adapt
Embrace the Tech: Institutions should invest in adopting and understanding AutoML tools and their applications. Regular training and workshops can be beneficial to this end.
Data Management: Implement efficient data management practices to maximize the utility of these tools. This includes practices to ensure data quality, security, and privacy.
Ethical Considerations: Financial institutions must adopt a holistic approach, considering ethical implications, including data privacy, bias, and fairness in automated decision-making.
Stay Informed: Keep up-to-date with the latest developments in AutoML to leverage its potential fully. Subscribe to tech news, research papers, and be part of relevant online communities.
IX. Conclusion
As we stand on the brink of an exciting new era, the convergence of finance and technology - particularly AutoML tools - is undeniable. This article has traversed the landscape of AutoML tools in finance, providing insights into their role, benefits, and the potential challenges they present.
A. Recap of Key Points
The Role of AutoML: AutoML tools have carved a niche in the finance sector due to their ability to automate complex tasks, reduce the need for expert human intervention, and provide high-quality predictive models.
Top Recommended Tools: Various AutoML tools, such as Google's AutoML, Microsoft's Azure AutoML, DataRobot, H2O.ai’s H2O AutoML, and RapidMiner Auto Model, have become popular for their unique features and advantages in the financial sector.
Emerging Tools: Emerging tools like Databricks AutoML, Neuraxle's AutoML, and MindsDB showcase the continuous evolution of AutoML.
Successful Application: AutoML has proven successful in diverse financial applications such as risk assessment, fraud detection, investment strategy formulation, and customer segmentation.
Ethical Considerations: With the promise of these tools also come challenges, including data privacy, regulatory compliance, and bias in automated decision-making.
The Future of AutoML: The future points towards wider adoption, greater integration, enhanced security, regulatory alignment, and increased explainability of AutoML tools.
B. Final Thoughts on the Use of AutoML in Finance
The road to embracing AutoML tools in finance requires embracing the tech, efficient data management, considering ethical implications, and staying updated with the latest developments. The key takeaway is that AutoML holds transformative potential for the finance sector, set to redefine traditional methods and drive innovation.
AutoML in finance is more than a trend; it's a revolution. Financial institutions that can adeptly harness its power will undoubtedly steer ahead, making more informed decisions, optimizing operations, and achieving a competitive edge. As we move forward, the focus should be on not just implementing but understanding these tools to adapt and evolve with them, shaping a smarter, more efficient future for finance.
X. Frequently Asked Questions (FAQs)
What are the best practices for implementing AutoML in finance?
Identify Business Needs: Before diving into AutoML, it's crucial to outline your business needs and align them with the capabilities of different tools.
Data Preparation: AutoML tools work best with well-structured, cleaned, and preprocessed data.
Continuous Learning and Adaptation: Technology and financial landscapes are always changing. It's necessary to keep up with new features, tools, and best practices.
Understand the Limitations: While AutoML tools are powerful, they are not silver bullets. Understanding their limitations will help you use them more effectively.
How secure are AutoML tools when handling sensitive financial data?
What are the costs associated with adopting AutoML tools in finance?
What roles can human data scientists play with the increase in AutoML adoption?
Can smaller financial institutions also benefit from AutoML tools? How?
How do AutoML tools improve fraud detection in finance?
Are there any downsides to using AutoML in finance?
How can AutoML help in customer segmentation?
What are the emerging trends in AutoML for finance beyond 2024?
How can AutoML support investment strategy formulation?
Comments