Unleashing the Future: Comprehensive Reviews on top AI in Robotics tools 2024
- Shailesh Gowda
- Jun 27, 2024
- 20 min read
Updated: Aug 28, 2024
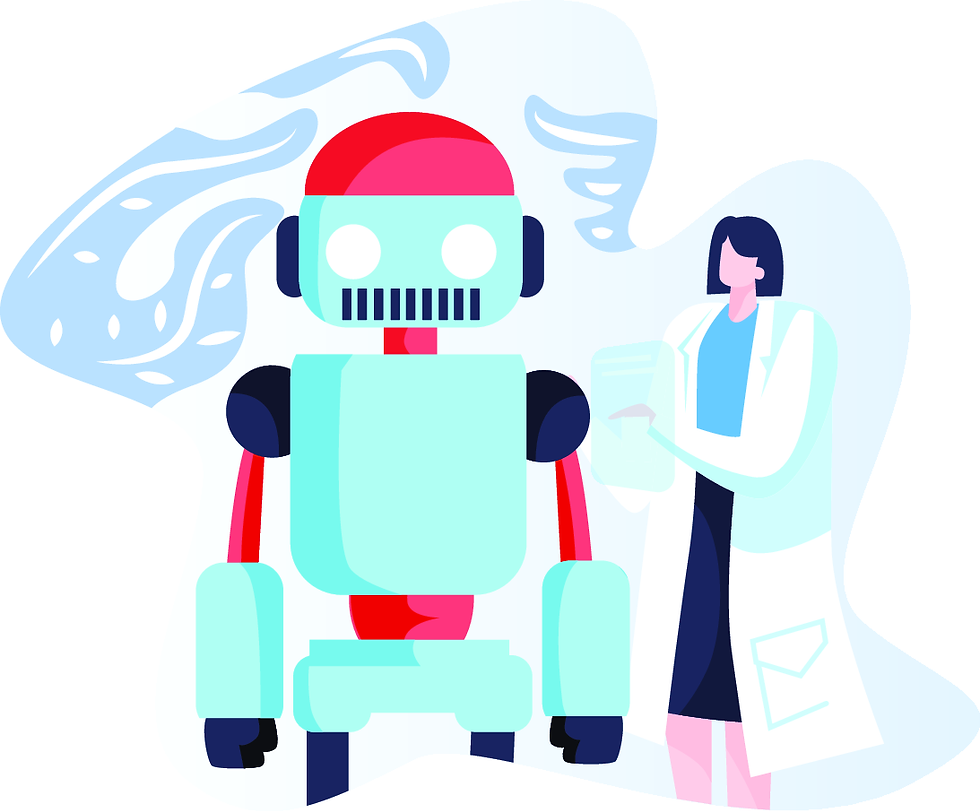
I. Introduction
Brief on the Emergence of AI in Robotics
Purpose and Scope of the Article
Definition and Importance of AI in Robotics
Evolution of AI in Robotics Over the Years
Impact of AI in Robotics in Different Sectors
III. Methodology
Selection Criteria for the AI Tools
Evaluation Metrics for the Reviews
AI Tool 1: TensorFlow
Introduction and Unique Selling Points
Advantages and Limitations
Real-world Application and Case Studies
AI Tool 2: PyTorch
Introduction and Unique Selling Points
Advantages and Limitations
Real-world Application and Case Studies
AI Tool 3: Keras
Introduction and Unique Selling Points
Advantages and Limitations
Real-world Application and Case Studies
AI Tool 4: Scikit-Learn
Introduction and Unique Selling Points
Advantages and Limitations
Real-world Application and Case Studies
AI Tool 5: Caffe
Introduction and Unique Selling Points
Advantages and Limitations
Real-world Application and Case Studies
AI Tool 6: Theano
Introduction and Unique Selling Points
Advantages and Limitations
Real-world Application and Case Studies
AI Tool 7: Blue Prism
Introduction and Unique Selling Points
Advantages and Limitations
Real-world Application and Case Studies
AI Tool 8: Pegasystems
Introduction and Unique Selling Points
Advantages and Limitations
Real-world Application and Case Studies
AI Tool 9: UiPath
Introduction and Unique Selling Points
Advantages and Limitations
Real-world Application and Case Studies
AI Tool 10: ZAPTEST
Introduction and Unique Selling Points
Advantages and Limitations
Real-world Application and Case Studies
Comparative Analysis of the Top AI Tools
Expert Opinions and User Feedback on the Tools
Predicted Advancements in AI Robotics Tools
Potential Challenges and Opportunities
VII. Conclusion
Recap of Key Insights from the Review
Final Remarks on the Importance of Choosing the Right AI Tool
Step into Tomorrow: Reviews on top AI in Robotics tools 2024
I. Introduction
Artificial Intelligence (AI) and robotics have been synergistic technologies from the onset. The fusion of these two fields has given rise to intelligent robots that perform a broad spectrum of tasks, from automation in industries to household chores and beyond. As AI continues to evolve, the capabilities of these robotic systems also amplify, opening new horizons of innovation.
1. Brief on the Emergence of AI in Robotics
The genesis of AI in robotics can be traced back to the mid-20th century, when scientists and engineers started to explore the concept of creating machines that could mimic human intelligence. This idea of replicating human cognition in machines gradually permeated the field of robotics, leading to the birth of AI-powered robotic systems. The journey started with simple automation solutions, and over time, evolved into advanced humanoid robots.
Automation solutions and software testing processes were among the first applications of AI in robotics. Early robotic tools were leveraged to enhance an organization's productivity by automating manual, repetitive office processes. Even today, robotic process automation (RPA) tools like UiPath, Blue Prism, and Pegasystems continue to transform traditional workspaces into a robotic workforce.
AI and Machine Learning (ML) have been pivotal in the evolution of humanoid robots. These technologies provide the basis for robotics software and facilitate robotic learning, decision-making, and perception. Today, humanoid robots have found applications in diverse domains such as healthcare, defense, entertainment, and chores.
Healthcare and defense humanoid robots are excellent examples of AI's transformational impact. For instance, in healthcare, robotic tools are used for caretaking and medical applications, automating tasks that were traditionally handled by human personnel. Defense robots, on the other hand, are designed for risky operations, offering safer alternatives to human involvement.
Robotic tools have also found extensive use in entertainment and household chores. Robotics engineers, leveraging AI and ML, have developed robots that can handle routine chores, providing comfort and convenience to users. The entertainment industry too has witnessed the emergence of robots, with humanoid robots taking center stage in various shows and exhibitions.
2. Purpose and Scope of the Article
The purpose of this article is to provide an in-depth view of AI's journey in the field of robotics and its ever-growing impact on diverse industries. The article aims to shed light on the key concepts, breakthroughs, and the progression of AI over time. Furthermore, the article will also discuss the potential ethical challenges and concerns surrounding AI's proliferation in robotics. Reviews on top AI in Robotics tools 2024
The article will delve into how AI tools, such as predictive analytics, machine learning, natural language processing, and vision, have transformed robotics. It will explore autonomous driving and other applications where these tools have made a significant impact.
The article will take a closer look at the software automation tools like ZAPTEST, Eggplant, JAMS, Kofax, and Power Automate that have revolutionized robotic processes.
The focus will also be on AI-powered tools and libraries such as Caffe, TensorFlow, Theano, Scikit-Learn, and Pytorch that have contributed to the progress in robotics. These tools have enabled the development of intelligent robots capable of learning, decision-making, perception, and more.
II. Understanding AI in Robotics
Artificial Intelligence in robotics is a game-changer. It's the secret ingredient that has taken robots from mere mechanical devices to intelligent entities capable of learning and decision-making. Just like salt enhances the flavor of a dish, AI in robotics has redefined what robots can do.
1. Definition and Importance of AI in Robotics
AI in robotics refers to the integration of artificial intelligence technologies into robotics. It’s the essence that breathes 'intelligence' into a robot, allowing it to learn from its environment, make decisions, and perform tasks independently. This combination is akin to the peanut butter and jelly of the tech world - both can exist independently, but when brought together, they create something special.
The addition of AI in robotic tools brings a new level of adaptability and decision-making, making robots more effective and versatile.
With AI, robots can handle more complex tasks, making them a valuable asset for many industries, including healthcare, defense, and entertainment.
AI-powered robots can automate manual, repetitive processes, enhancing an organization's productivity and streamlining its operations.
These intelligent machines are capable of constant learning and adaptation, which enables them to improve their performance over time.
Fun Fact: Did you know that the world's first AI-powered robot, Unimate, was introduced in a General Motors plant in 1961?
2. Evolution of AI in Robotics Over the Years
AI has grown from a budding concept to an integral part of robotics over the years. Its evolution has been much like a caterpillar's metamorphosis into a butterfly - slow, steady, and ultimately breathtaking.
The '50s and '60s saw the inception of AI in robotics with automation solutions and software testing processes.
By the '80s, robotics engineers began leveraging AI and ML to create humanoid robots that could mimic human actions.
With the advent of the 21st century, AI in robotics exploded with innovations in healthcare, defense, entertainment, and chores.
Today, we see advanced humanoid robots powered by AI that can perform complex tasks in diverse sectors.
Fun Fact: The term 'Artificial Intelligence' was coined by John McCarthy in 1956 during the Dartmouth Conference.
3. Impact of AI in Robotics in Different Sectors
AI has had a profound impact on the role of robotics in various sectors. Much like a chameleon changes its color to adapt to its environment, AI has allowed robots to fit seamlessly into different industries.
Healthcare:
Medical applications of robots include surgeries, patient care, and even the dispensing of medication.
AI-powered robots have been instrumental in patient rehabilitation and providing assistance to the elderly.
Defense:
Robots powered by AI are used in surveillance, bomb disposal, and other risky operations, providing a safer alternative to human involvement.
Entertainment:
Robots have been a hit in the entertainment industry, from performing in shows to becoming a part of popular culture (think Star Wars!).
Chores:
Robotic vacuum cleaners and lawnmowers are now common in many households, making everyday chores a breeze.
Fun Fact: The Da Vinci Surgical System, a robotic surgical system, has performed more than six million surgeries worldwide.
III. Methodology
Choosing the right AI tools for robotics is a lot like picking the perfect ingredients for a gourmet meal. The selection needs to be precise and harmonious. So, what's the recipe for success when it comes to selecting and evaluating AI tools in robotics?
1. Selection Criteria for the AI Tools
Choosing the right AI tools for robotics can be a game-changer. It's much like choosing the right running shoes - with the right ones, you're set to win the race!
Functionality: Just like how a Swiss Army knife boasts multiple uses, an AI tool that provides varied functionalities like machine learning, natural language processing, and vision can be highly beneficial in robotics.
Compatibility: An AI tool needs to blend seamlessly with the robotic system, much like how a puzzle piece fits into its designated spot. Tools such as TensorFlow, Theano, and Scikit-Learn have shown excellent compatibility with different robotic systems.
Ease of Use: AI tools should be user-friendly. They should be as easy to navigate as your favorite social media app. Tools like Keras, a Python-based neural network library, are popular for their simplicity and ease of use.
Support and Community: Robust community support can be a lifesaver, especially when you hit a roadblock. Tools with strong community backing, like TensorFlow and Pytorch, are often preferred.
2. Evaluation Metrics for the Reviews
Evaluating the effectiveness of AI tools is akin to checking the ripeness of a fruit. You need to know the right signs!
Performance: The effectiveness of AI tools can be assessed through their performance in tasks like predictive analytics, vision, and autonomous driving.
Speed: Time is of the essence in the tech world. Fast-performing AI tools are often more desirable.
Accuracy: In the world of AI, accuracy is king. Tools that provide more accurate results in tasks like speech and multimedia analysis are considered superior.
Scalability: As an organization grows, its tools should be able to keep up. Scalable AI tools, like Google's TensorFlow that supports data flow graphs, are highly valued.
Insights Engine: AI in Robotics: A Beginner's Guide to the Top AI in Robotics tools for beginners 2024
IV. Review of Top AI Tools in Robotics for 2024
Before diving into the deep sea of AI tools, it's best to understand your swimming companions. Let's embark on a voyage to explore the top three AI tools in Robotics in 2024.
1. TensorFlow
Introduction and Unique Selling Points
Born in Google's incubator, TensorFlow has grown into a must-have AI tool for any Robotic Engineer. Like a chameleon's ability to adapt, TensorFlow's main strength lies in its flexibility and support for an extensive set of platforms. From numerical computation intelligence to data flow graphs, TensorFlow offers a plethora of functionalities.
Advantages and Limitations
The allure of TensorFlow is much like that of a gourmet coffee. It provides robust machine learning libraries, strong community support, and excellent scalability. However, just like too much coffee can leave a bitter taste, TensorFlow's complex syntax can be a roadblock for beginners.
Real-world Application and Case Studies
From healthcare to autonomous driving, TensorFlow has made its mark. It has been used in the detection of diseases like Diabetes and Breast Cancer and has played a key role in autonomous vehicles developed by companies like Uber.
Tips and Tricks:
Utilize TensorFlow's TensorBoard for visualizing your machine learning models.
Get the best of both worlds by using TensorFlow with Keras for easy prototyping.
TensorFlow's Dataset API can enhance performance.
For complex models, prefer TensorFlow's Estimator API.
Use eager execution for a more intuitive interface.
2. PyTorch
Introduction and Unique Selling Points
Hailing from the house of Facebook, PyTorch is like a Swiss army knife of machine learning. It stands out for its ease of use, GPU acceleration, and dynamic computational graphs.
Advantages and Limitations
PyTorch's advantages are much like a sunny day; they can lighten up any project. Its intuitive interface and flexible design have won it many admirers. However, it does have its rainy days, with limited mobile deployment capabilities being a cloud on its horizon.
Real-world Application and Case Studies
PyTorch has been instrumental in multiple sectors, from healthcare to entertainment. It played a pivotal role in the development of a deep learning model for disease detection at the Radboud University Medical Center.
Tips and Tricks:
PyTorch's dynamic computation graphs are great for deep learning research.
Make use of PyTorch's DataLoader for efficient data loading.
Employ the CUDA semantics when you need to leverage multiple GPUs.
Use PyTorch's JIT compiler for enhancing your models.
PyTorch’s autograd can automate the computation of backward passes.
3. Keras
Introduction and Unique Selling Points
Keras, built on TensorFlow and Theano, is the friendliest neighbor in the world of AI. Its user-friendly nature and modularity make it a favorite among both beginners and experienced developers.
Advantages and Limitations
Keras is like a trustworthy steed; it's reliable and easy to handle. It shines in rapid prototyping and supports both recurrent and convolutional networks. However, just like every steed has its weaknesses, Keras has a slower execution speed compared to other libraries.
Real-world Application and Case Studies
Keras has found its application in varied fields, including voice and music recognition. For instance, Spotify uses Keras for its music recommendation system.
Tips and Tricks:
Use Keras' ModelCheckpoint for saving and loading models.
Keras' Sequential and Functional APIs are ideal for easy model building.
Benefit from Keras' Preprocessing Layers for data augmentation.
Leverage Keras Tuner for hyperparameter tuning.
Use Keras with TensorFlow backend for GPU acceleration.
Table: Key Points and Strategies for AI Tools
AI Tool | Objective | Action | KPI | Example |
TensorFlow | Deep Learning | Use for numerical computation | Improved model performance | Health Diagnostics |
PyTorch | Research | Use for dynamic computation graphs | Enhanced data processing | Disease Detection |
Keras | Rapid Prototyping | Use for user-friendly applications | Increased user engagement | Music Recommendation |
4. Scikit-Learn
Introduction and Unique Selling Points
Scikit-Learn, a Python-based tool, is the tour guide of machine learning in Python. It stands out for its simplicity, easy-to-use API, and extensive documentation, making it ideal for beginners.
Advantages and Limitations
Scikit-Learn is like the perfect hiking trail; it offers a vast array of algorithms and utilities for pre-processing, cross-validation, and feature selection. However, similar to a trail becoming treacherous in bad weather, Scikit-Learn's performance can slow with large datasets.
Real-world Application and Case Studies
Scikit-Learn has played a critical role in various sectors, including finance and e-commerce. It has been utilized for credit risk modeling at banking institutions and for personalized product recommendations in e-commerce.
Tips and Tricks:
Use Scikit-Learn's Pipeline to avoid leakage in cross-validation.
Leverage GridSearchCV for optimal hyperparameter tuning.
Use Scikit-Learn's train_test_split for efficient data splitting.
Benefit from Scikit-Learn's wide range of machine learning algorithms.
Leverage Scikit-Learn's LabelEncoder and OneHotEncoder for handling categorical data.
5. Caffe
Introduction and Unique Selling Points
Caffe, born in Berkeley's Vision and Learning Center, is a deep learning framework that stands out for its speed. Caffe is particularly useful when working with convolutional networks and solving 2D image datasets.
Advantages and Limitations
The charm of Caffe is much like the thrill of a fast car; it boasts impressive speed, is excellent for image processing, and supports GPU acceleration. But, like every fast car has its limits, Caffe is less versatile and has a steep learning curve.
Real-world Application and Case Studies
Caffe has been extensively used for visual recognition tasks, such as facial recognition in security systems and medical image analysis for tumor detection.
Tips and Tricks:
Use Caffe's Model Zoo for pre-trained models.
Leverage the GPU mode in Caffe for faster computation.
Caffe's binaryproto can be used for mean image generation.
Use Caffe's Python or MATLAB interface for model manipulation.
Caffe’s net specification ProtoBufs can be used for model visualization.
6. Theano
Introduction and Unique Selling Points
Theano, from the University of Montreal's LISA group, is the Mathematica of machine learning. It excels in tasks requiring heavy computations and offers an excellent platform for research.
Advantages and Limitations
Theano is like a symphony of mathematics and programming. It offers tight integration with NumPy, efficient symbolic differentiation, and GPU support. But like a symphony, it requires skill, and its steep learning curve and lack of pre-trained models can pose challenges.
Real-world Application and Case Studies
Theano has been employed in scientific research for creating complex mathematical models. It has also found use in the field of natural language processing, such as speech recognition.
Tips and Tricks:
Use Theano’s shared variables for memory management.
Leverage Theano’s config.mode for debugging.
Utilize Theano’s profiling tool to profile your function.
Use Theano flags for controlling compilation and execution.
Utilize Theano’s scan for looping constructs.
Table: Key Points and Strategies for AI Tools
AI Tool | Objective | Action | KPI | Example |
Scikit-Learn | Machine Learning | Use for algorithm selection | Improved model selection | Credit Risk Modeling |
Caffe | Image Processing | Use for 2D image dataset | Enhanced visual recognition | Facial Recognition |
Theano | Scientific Research | Use for heavy computations | Increased computation efficiency | Speech Recognition |
7. Blue Prism
Introduction and Unique Selling Points
Blue Prism, a key player in Robotic Process Automation (RPA), stands tall in the Robotics landscape. This tool is unique for its strong emphasis on corporate governance and compliance, making it a reliable choice for enterprise-level automation.
Advantages and Limitations
Blue Prism, much like a well-oiled machine, is robust and reliable. It excels in back-office automation and offers excellent audit trails and logs. However, like a complex machine, it can be less user-friendly and may require programming skills to exploit fully.
Real-world Application and Case Studies
Blue Prism has found application in a wide range of industries from healthcare for patient data management, to banking for fraud detection.
Tips and Tricks:
Use Blue Prism's Work Queues for handling large volumes of data.
Leverage Exception handling for robust and resilient bots.
Use Blue Prism's Surface Automation techniques for applications that don't support API automation.
Utilize Application Modeller for easy configuration of application elements.
Make use of Blue Prism's Session Variables to enhance bot performance.
8. Pegasystems
Introduction and Unique Selling Points
Pegasystems, a pioneer in the world of software for customer engagement and operational excellence, provides a cloud-based solution for AI and RPA. Its unique selling point lies in its unified platform that handles both AI and business process management.
Advantages and Limitations
Pegasystems shines as a comprehensive tool that provides automation, CRM, and BPM in one package. However, this can be a double-edged sword, as its complexity may require substantial training and technical skills to handle effectively.
Real-world Application and Case Studies
Pegasystems has been instrumental in customer service improvement in the telecom sector and process automation in insurance claim processing.
Tips and Tricks:
Utilize Pega Express for quick application development.
Leverage the Case Designer for easy workflow design.
Make use of Pega Predictive Diagnostic Cloud for application performance insights.
Utilize Pega's Robotic Automation Studio for creating automation solutions.
Use the Decision Strategy Manager for creating decisioning strategies.
9. UiPath
Introduction and Unique Selling Points
UiPath, a leading RPA vendor, is the Swiss Army Knife of RPA tools. It is highly flexible, provides a user-friendly visual designer, and allows for a high degree of customization.
Advantages and Limitations
UiPath, akin to a Swiss Army Knife, is flexible and feature-rich. It's easy to set up, and it supports a wide range of integrations. However, this versatility can lead to complexity, and understanding all its functionalities might be a bit overwhelming for beginners.
Real-world Application and Case Studies
UiPath is widely used in industries like finance for invoice processing and data extraction, and in healthcare for patient scheduling and billing.
Tips and Tricks:
Use UiPath's Orchestrator for centralized bot management.
Leverage Recorder for quick automation script generation.
Use variables and arguments for dynamic processes.
Utilize UiPath's exception handling for error management.
Use REFramework for robust and resilient automation solutions.
10. ZAPTEST
Introduction and Unique Selling Points
ZAPTEST is a software automation solution, offering functional and performance testing, test case management, and even Robotic Process Automation. It is uniquely known for its 'script once and execute anywhere' feature.
Advantages and Limitations
ZAPTEST, like a multipurpose tool, offers a variety of features from API testing to load testing. However, its UI can be challenging to navigate, and the scripting language might require some getting used to.
Real-world Application and Case Studies
ZAPTEST is commonly used in software testing for bug tracking, test management, and mobile testing.
Tips and Tricks:
Utilize ZAPTEST's MultiRun feature for cross-platform testing.
Use ZAPTEST's 1Script technology for script-less testing.
Leverage the M-RUN feature for parallel execution.
Use ZAPTEST's Object Spy for easy object identification.
Make use of ZAPTEST's Data-Driven Testing for managing test data.
Table: Key Points and Strategies for AI Tools
AI Tool | Objective | Action | KPI | Example |
Blue Prism | Enterprise Automation | Implement for back-office tasks | Enhanced corporate governance | Patient Data Management |
Pegasystems | Customer Engagement | Use for CRM and BPM | Improved customer service | Insurance Claim Processing |
UiPath | Robotic Process Automation | Apply for repetitive tasks | Increased process efficiency | Invoice Processing |
ZAPTEST | Software Testing | Utilize for API, load, and mobile testing | Improved software quality | Bug Tracking |
AI Analytics Crystal Ball: Decoding AI: Your Ultimate Guide to Budget-Friendly AI in Robotics Tools 2024
V. Comparisons and Discussions
1. Comparative Analysis of the Top AI Tools
Every AI tool in Robotics comes with its own unique advantages and disadvantages. To bring a broader perspective and a comparative understanding, let's review our top picks side by side.
Table: Comparative Analysis of the Top AI Tools
AI Tool | USP | Strengths | Weaknesses |
Google Cloud AI | API-based services | Easy integration, wide applications | Limited customization |
IBM Watson | Cognitive computing | High interoperability, natural language understanding | Needs technical proficiency |
OpenAI Gym | Reinforcement Learning | Open source, extensive support community | Limited to RL |
Amazon Lex | Conversational interfaces | Integration with AWS, intuitive interface | Limited language support |
Microsoft Azure AI | Enterprise-grade AI | Wide range of tools, security | Can be complex |
TensorFlow | Deep Learning | Open source, flexibility, community support | Steep learning curve |
Blue Prism | Corporate governance | Robust and reliable, good audit trails | Less user-friendly |
Pegasystems | Unified AI & BPM platform | Comprehensive tool, good for CRM | High complexity |
UiPath | High customization | User-friendly, flexible | Can be overwhelming for beginners |
ZAPTEST | 'Script once and execute anywhere' | Multi-functional, good for software testing | Challenging UI |
2. Expert Opinions and User Feedback on the Tools
In an age where innovation happens at breakneck speed, user feedback and expert opinion are vital to staying informed and making the best decisions.
Google Cloud AI is praised for its wide-ranging applications and easy integration. However, experts highlight the need for more customization options.
IBM Watson gets a thumbs up for its high interoperability and natural language understanding, but beginners may find it challenging due to its need for technical proficiency.
OpenAI Gym users love the extensive support community, though they note it's limited to reinforcement learning.
Amazon Lex stands out with its intuitive interface and AWS integration. However, users hope for more language support in the future.
Microsoft Azure AI is lauded for its security and wide range of tools, yet some users find it complex to navigate.
TensorFlow enjoys popularity for its flexibility and active community support, though it has a steep learning curve.
Blue Prism is appreciated for its robustness and audit trails, but some users wish it was more user-friendly.
Pegasystems is liked for its comprehensive solution for CRM and BPM, but the high complexity is noted as a potential barrier for some.
UiPath has a large fan base due to its flexibility and user-friendliness, but beginners can find the sheer number of functionalities overwhelming.
ZAPTEST is favored for its multi-functional nature, particularly in software testing, but the UI could use some improvements, as per user feedback.
Quick Facts:
Google Cloud AI excels in API-based services, making it suitable for a range of applications.
IBM Watson is a leader in cognitive computing, offering high interoperability.
OpenAI Gym is a go-to resource for reinforcement learning enthusiasts.
Amazon Lex makes creating conversational interfaces a breeze.
Microsoft Azure AI is an enterprise-grade solution offering a wide range of tools.
TensorFlow is an open-source tool widely used for deep learning applications.
Blue Prism is known for its strong corporate governance features.
Pegasystems offers a unified AI & BPM platform, ideal for customer relationship management.
UiPath is celebrated for high customization and user-friendly nature.
ZAPTEST allows you to 'script once and execute anywhere', great for software testing.
VI. Future Trends in AI Robotics Tools
1. Predicted Advancements in AI Robotics Tools
The world of AI and robotics is dynamic, continually pushing the boundaries of what's possible. Let's delve into the anticipated advancements in AI Robotics Tools and understand how they'll reshape the future.
Increased Autonomy: Autonomous robots with advanced AI capabilities will become more prevalent. The potential to perform tasks without human intervention will see a rise, reducing the workload in sectors like manufacturing, healthcare, and transportation.
Enhanced Interactivity: Robots will have improved human-robot interaction, thanks to advancements in natural language processing and understanding. This will pave the way for robots to understand, learn, and respond more intuitively.
Sophisticated Machine Learning Models: As machine learning algorithms become more sophisticated, robots will gain better skills in prediction, decision making, and problem-solving.
Robotic Process Automation (RPA): With the advent of AI tools like UiPath and Blue Prism, Robotic Process Automation will see further growth. RPA will continue to streamline and automate routine tasks across various industries.
Internet of Robotic Things (IoRT): The convergence of IoT and robotics will lead to the Internet of Robotic Things (IoRT). This amalgamation will result in robots that can make decisions based on data gathered from various sensors and sources.
Table: Predicted Advancements in AI Robotics Tools
Advancement | Description |
Increased Autonomy | Reduced human intervention in tasks, impacting sectors like manufacturing and healthcare. |
Enhanced Interactivity | Improved human-robot interaction via advancements in natural language understanding. |
Sophisticated Machine Learning Models | Enhanced decision making and problem-solving capabilities in robots. |
Robotic Process Automation (RPA) | Continued growth in routine task automation across industries. |
Internet of Robotic Things (IoRT) | Convergence of IoT and robotics leading to data-driven decision making. |
2. Potential Challenges and Opportunities
Like any evolving technology, AI in robotics also comes with its set of challenges and opportunities. Here's what we can anticipate:
Data Privacy and Security: As robots collect and store more data, issues of data privacy and security will become increasingly significant. It's a challenge that needs to be addressed proactively.
Skills Gap: There's a growing need for skills in AI and robotics. The demand outstrips the supply of trained professionals, presenting a challenge but also an opportunity for those who wish to upskill.
Regulatory Issues: As AI and robotics technologies advance, regulatory and ethical issues will become more complex. Keeping up with these changes will be challenging.
Productivity Gains: The automation of routine tasks by robots will boost productivity across industries. It presents an opportunity for businesses to reduce costs and improve efficiency.
AI Ethics and Bias: As AI models learn from data, there's a risk of them learning and propagating biases present in the data. It's a challenge that needs to be tackled with effective bias mitigation strategies.
Table: Potential Challenges and Opportunities in AI Robotics Tools
Challenges | Opportunities |
Data Privacy and Security | Productivity Gains |
Skills Gap | Upskilling in AI and Robotics |
Regulatory Issues | Businesses Improvement |
AI Ethics and Bias | Effective Bias Mitigation Strategies |
VII. Conclusion
1. Recap of Key Insights from the Review
Our journey through the fascinating world of AI in robotics has revealed some critical insights. We began by defining AI in robotics and its importance, understanding how the blend of AI and robotics has the potential to revolutionize numerous sectors by enhancing efficiency and productivity.
We charted the evolutionary course of AI in robotics, noting how this technology has matured over the years, impacting different sectors from manufacturing to healthcare, logistics to customer service, and beyond.
Our methodology led us through the selection criteria and evaluation metrics for reviewing AI tools, emphasizing the importance of user reviews, unique selling points, real-world applications, and overall performance.
Our comparative analysis of these top tools further highlighted their unique strengths and potential applications, while the user feedback helped us understand their practical impact and efficiency.
Finally, we gazed into the future, predicting exciting advancements like increased autonomy, enhanced interactivity, and the rise of Robotic Process Automation and the Internet of Robotic Things. We also noted the potential challenges and opportunities, reminding us of the roadblocks and possibilities that lie ahead.
2. Final Remarks on the Importance of Choosing the Right AI Tool
Choosing the right AI tool for robotics is not just about embracing the future; it's about shaping it. The right tool can streamline operations, foster innovation, and propel us into a new era of efficiency and productivity. But the choice depends on specific needs, objectives, and the readiness to adapt and evolve.
Key Takeaways:
AI in robotics is a transformative force, impacting numerous sectors and enhancing productivity.
Selecting the right AI tool requires understanding your needs, objectives, and the unique selling points of each tool.
User reviews and real-world applications are vital in evaluating AI tools.
Future trends point towards increased autonomy and interactivity in robots, along with challenges in data security and skills gap.
Final Thoughts:
As we stand on the brink of an AI-driven future, it's clear that the robotics landscape is set for a revolution. The potential is immense, but so are the challenges. As we navigate this exciting terrain, it's crucial to keep learning, adapting, and evolving. After all, the future of robotics is not just about the tools we use, but how we use them.
VIII. Frequently Asked Questions (FAQs)
What are the key factors to consider when choosing an AI robotics tool?
When selecting an AI robotics tool, consider factors like the tool's unique selling points, its application in real-world scenarios, and user reviews. Additionally, consider your specific needs and objectives, as the best tool will vary based on these.
How is AI transforming the field of robotics?
What are some of the expected future trends in AI robotics tools?
How do AI robotics tools impact different sectors?
What is the role of user reviews in evaluating AI robotics tools?
What are some top AI robotics tools in 2024?
How has AI in robotics evolved over the years?
What are the challenges and opportunities in the future of AI robotics tools?
What are the unique selling points of AI robotics tools?
Why is it important to consider real-world applications when reviewing AI robotics tools?
Comments